_______________________________
8:02 PM
8:02 PM
What better way to close out the live blog than with this tweet? Thanks again to everyone who attended, spoke, found inspiration, and followed along.
_______________________________
6:14 PM
6:14 PM
More photos from the re:MARS party - Furrion Exo-Bionics Mech, pace car laps, Segway course, astronaut photo op, and more.
01 / 08
_______________________________
3:08 PM
3:08 PM
Scenes from the All Stars BattleBots challenge at last night's re:MARS party.
01 / 05
_______________________________
12:30 PM
12:30 PM
More photos and details to come, but our last re:MARS sessions have come to an end. A huge thanks to all attendees, speakers, and vendors, as well as those who followed along with the live blog.
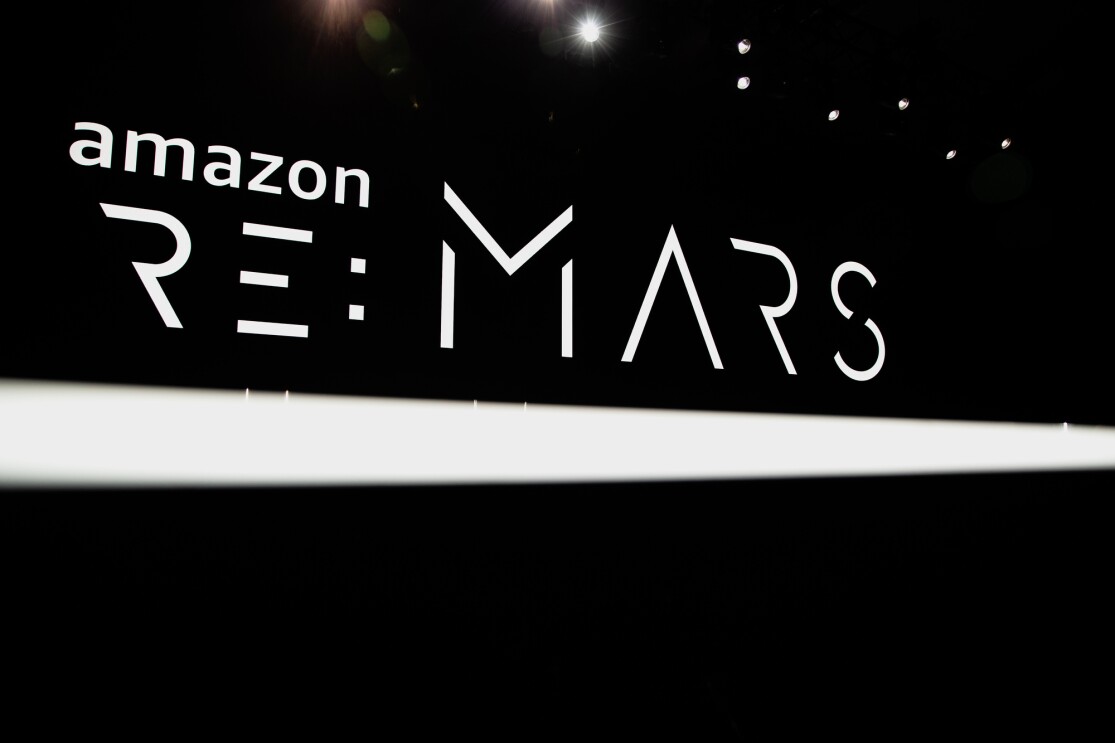
_______________________________
11:35 AM
_______________________________
9:49 AM
9:49 AM
"Is it the algorithm that needs to be ethical, or it is the people, processes and systems that should be ethical? - Dr. Prem Natarajan
Algorithms are not moral agents, but ultimately the humans who design and deploy them are several degrees separated from the algorithm's decision making, so they may inadvertently introduce bias." - Dr. Aaron Roth
"That doesn't make it okay. The algorithm is kicking women out of the employment system, incarcerating people of color. We have to come up with "responsible AI." - Dr. Nicol Turner-Lee
_______________________________
9:41 AM
9:41 AM
"It's important for government to not over-regulate, but also important to have places and spaces to have anti-bias experiments."
_______________________________
9:32 AM
9:32 AM
"is it fair to charge teen male drivers more for car insurance, because they statistically have more accidents? Or is it more fair to charge teen female drivers and males the same, so the females subsidize the cost? - Fairness varies depending on the parties involved."
_______________________________
9:29 AM
9:29 AM
"Sometimes there isn't a definition of fairness that will be fair for everyone."
_______________________________
9:22 AM
9:22 AM
"The goal is to create a ML model that minimizes classification error (example: a group of students who might use a SAT tutor before taking the test, and another population who wouldn't work with tutors before taking the test). A model that is designed to fit the majority population, it will introduce bias against a minority population, because fitting the model to that population would not fit the majority population. Even without race considered, bias is introduced."
_______________________________
9:14 AM
9:14 AM
"AI can shine a light on specific biases."
_______________________________
9:12 AM
9:12 AM
"It's important for government to assess - Is this an area we want to automate, and if we automate, are we considering the entire ecology when placing the model, as there are existing biases in the system?"
(in reference to using AI to predict whether people will appear at court for summons - studies have shown that the primary reason individuals miss court is due to lack of childcare or transportation, not direct avoidance of summons)
_______________________________
9:06 AM
9:06 AM
Panel: AI for everyone: promoting fairness in ethical AI
"Requires partnership between nonprofits, industry, government, and academia in order for AI to work for everyone."
_______________________________
8:42 AM
8:42 AM
Leo Jean Baptiste explores re:MARS with us.
01 / 04
_______________________________
6:19 AM
06/07/19
6:19 AM
06/07/19
It's the final day of re:MARS 2019, we've got a few breakout sessions remaining, and so much more to share, right here.
_______________________________
11:33 PM
11:33 PM
We're calling it a night. We'll be back tomorrow morning with sessions, recaps, and more.
_______________________________
10:06 PM
10:06 PM
Nite Wave performing at re:MARS.
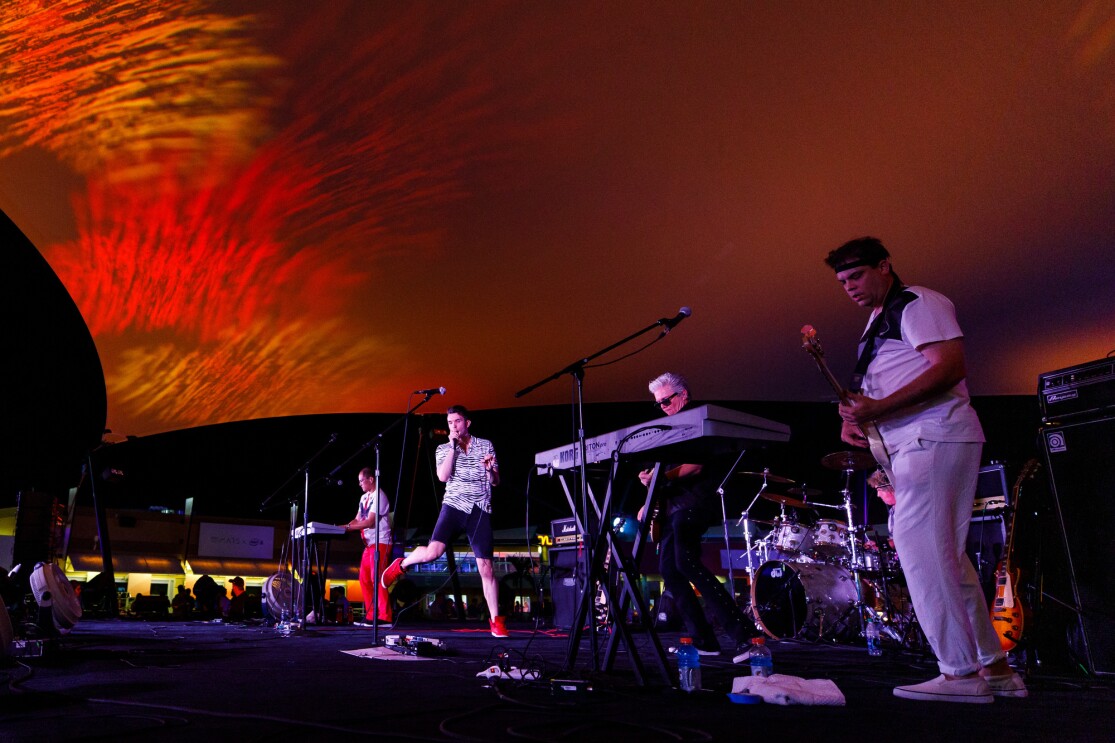
_______________________________
9:07 PM
9:07 PM
The re:MARS party is in full swing. Currently watching BattleBots, livestreaming on Twitch.
_______________________________
6:21 PM
6:21 PM
_______________________________
5:46 PM
5:46 PM
Jeff Bezos' fireside chat with Jenny Freshwater.
_______________________________
5:41 PM
5:41 PM
In a breakout session, Andrew Lafranchise - senior software development engineer, Amazon Web Services, and Alejandra Olvera-Novack - AWS developer relations, Amazon Web Services teach attendees how to build a simulation of a TurtleBot – a low-cost, personal robot kit with open-source software. Using AWS Robomaker, robot developers can leverage AWS machine learning services, monitoring services, and analytics services to enable their robot to stream data, communicate, comprehend, and learn how to navigate new environments.
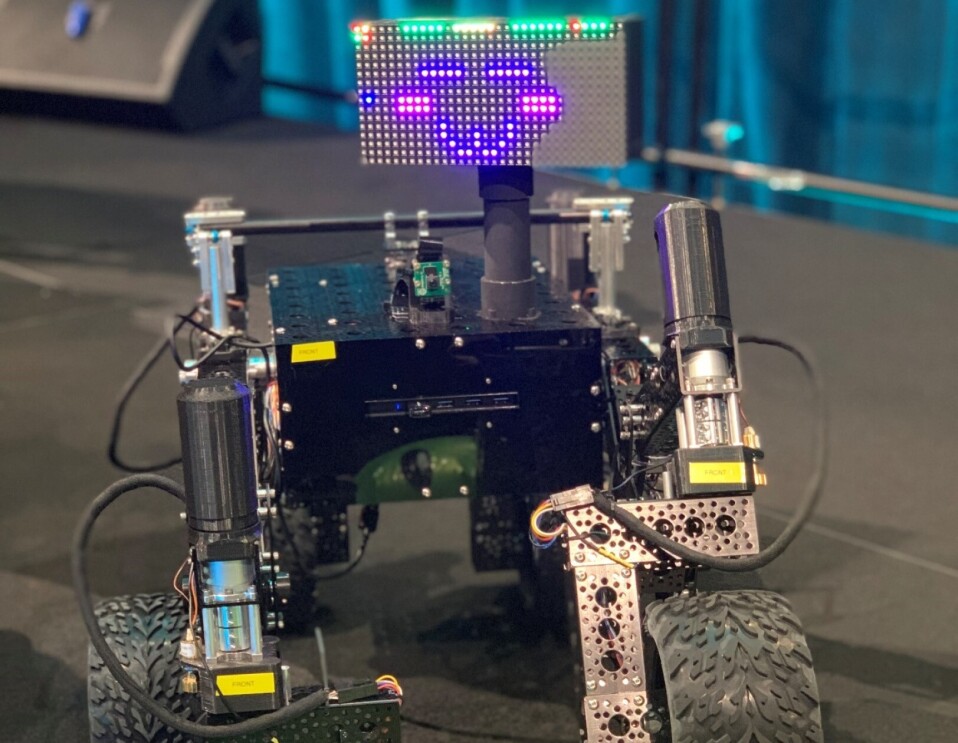
_______________________________
5:22 PM
5:22 PM
Breakout session about how machine learning can detect emotions in a speaker's voice, hosted by Chao Wang, senior manager, applied science, Amazon and Viktor Rozgic, senior applied scientist, Alexa Speech at Amazon.
While human emotions are complicated, they can be represented as feature vectors by mapping them on a three dimensional axis. A feature vector is a representation of an object’s characteristics in numbers – this allows an algorithm to measure properties of an object. For detecting emotion, Alexa machine learning scientists mapped utterances on three dimensional axes – valence (negative – positive), activation (the intensity of the emotion from low to high) and dominance (a measure of submissiveness). For example, anger would be negative on the valence scale, positive on the activation scale and positive on the dominance scale.
Visual:
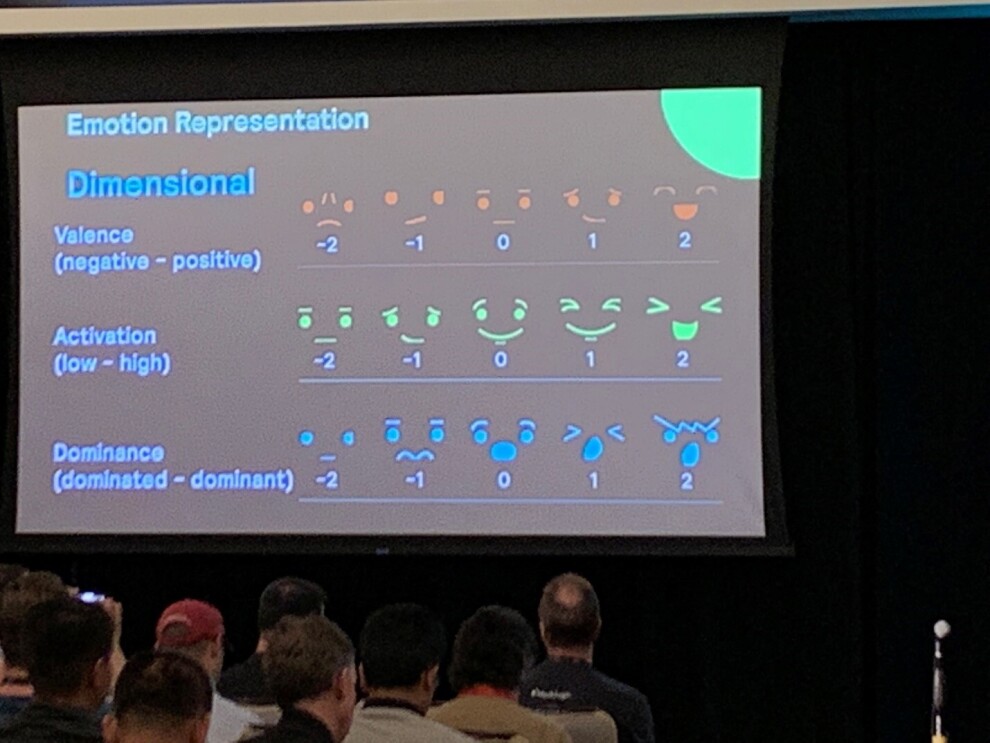
_______________________________
5:00 PM
5:00 PM
_______________________________
4:45 PM
4:45 PM
Why we should share with others:
When people share enthusiasm and excitement, it can trip that part in the back of our minds, to think 'I might be able to contribute to that.
_______________________________
4:42 PM
4:42 PM
"In life and work, mindset is primary, training is secondary."
_______________________________
4:22 PM
4:22 PM
When delegating: "You have to give everyone complete autonomy within a tiny bandwidth. When you've got someone you trust, their work is going to add value. I don't get mad at the people who get it wrong, I just move on to the person who gets it right. It's a process, I'm still learning it."
_______________________________
4:17 PM
4:17 PM
"I've given some talks at TED, I remind myself that "this is only the next 20 minutes," but yes, I consider the long tail impact and my personal values of sharing, love, inclusivity, and being a participant in a culture."
_______________________________
4:06 PM
4:06 PM
Balancing the technical and creative mind
"We say "it's an art and a science," so we're putting them at different ends of the spectrum - that's a disservice. Forming a hypothesis is a deeply creative act. Engineering is a deeply creative field, there are many ways to solve a problem, just like a painting."
_______________________________
4:03 PM
_______________________________
4:03 PM
How to quiet negative speak
"Time helps me quiet it, nothing else works or helps me stop my mind from insulting me. I don't know how to stop my inner critic, but if I talk about it with my partner and friends, it takes away some of its power, and it helps others, too. There's no future where stuff gets easier."
_______________________________
3:59 PM
_______________________________
3:59 PM
"It's vital to reach out to marginalized communities to ensure they have access to the same stuff."
_______________________________
3:58 PM
_______________________________
3:58 PM
"Always add "A" to STEM - a great idea isn't a great idea until you can communicate it to someone, that's art."
_______________________________
3:56 PM
_______________________________
3:56 PM
"Everything that's great came about because someone was obsessed about it."
_______________________________
3:55 PM
_______________________________
3:55 PM
Making can be anything. Dress making, welding, coding.
"Those things we can't stop paying attention to are where we find our excellence."
_______________________________
3:54 PM
3:54 PM
"We love the myth of the singular creator. They're amazing human beings, but they're surpassingly rare. We have to work together.
Sharing is so important. Sharing credit, joy, enthusiasm, work. A tiny bit of encouragement meant so much to me. It really mattered, and I saw how important it was to share encouragement with others - and to share knowledge, just to share. It incurs no cost. Every bit you share on a personal level pays huge dividends."
_______________________________
3:49 PM
3:49 PM
"Science and art are two different ways of telling the same stories."
_______________________________
3:49 PM
3:49 PM
"Over the 13 years I was on Mythbusters, I learned every skill on the job."
_______________________________
3:48 PM
_______________________________
3:48 PM
"We need to normalize iterations, for kids especially, to show that iterations are a part of the process."
_______________________________
3:44 PM
3:44 PM
"I didn't expect to be so philosophical in the book."
On momentum:
What helps me have momentum is obsession. Is this something I need to see through fruition. Even if I have to scrap everything and start from scratch.
What helps me have momentum is obsession. Is this something I need to see through fruition. Even if I have to scrap everything and start from scratch.
I use checkboxes, I love to color them in. In fact I create other boxes like "drive to work, eat a sandwich" because it's so satisfying.
Applying tolerance to self:
I still hit snags, but I get to talk about them. Not only do i hit snags, but my inner critic is super harsh. On a monthly basis, my inner critic says "you have no right to make things, you should quit now"
I still hit snags, but I get to talk about them. Not only do i hit snags, but my inner critic is super harsh. On a monthly basis, my inner critic says "you have no right to make things, you should quit now"
_______________________________
3:41 PM
3:41 PM
Talking about human creativity, in relation to his new book, "Every tool is a hammer."
"You have to get it done. Nobody needs to know why, you just have to do it.
The film industry has stupid-tight deadlines. And I love that those deadlines help you determine where your tolerances are, and how much energy you put into that thing - the tolerance of acceptance."
_______________________________
3:39 PM
3:39 PM
"I got to play around with the haptic arms. Every once in a while I get to play with a technology that feels like my fantasies. That was it."
_______________________________
3:33 PM
3:33 PM
Adam Savage is speaking on stage.
"I have a show coming out on Discovery Channel, called "Savage Builds" - it's an absurd engineering show."
_______________________________
2:30 PM
2:30 PM
From re:MARS keynote with Marc Raibert, CEO and founder Boston Dynamics
01 / 04
Three robots at Boston Dynamics. Handle, which is a purpose built robot, it's really designed to just do one simple set of tasks. The second robot is Spot, which is a general purpose robot. It's been designed as a mobility platform that can be customized for a wide variety of applications. And then thirdly Atlas, our r&d robot. Atlas is like the Lamborghini or the Maserati of robots: really expensive, really finicky needs a whole team to keep working, but delivers the highest levels of performance.
People have a great ability to stabilize their hands when their body moves. And that means you can get mobile manipulation that's very effective. And that's what we sought to emulate with Spot.
There's a traditional understanding for how robots work. There's a computer and software that listen to the sensors from the robot and tell the robot what to do. But that's only half of the story. And the other half is that there's a physical world out there, that's also telling the robot what to do, gravity's pulling on it, friction is retarding its motions, energy that's stored in compliance is or the motion of the robot.
You really want to take a holistic approach, and combine what the software is doing with the physical machine and its design.
_______________________________
1:54 PM
1:54 PM
"The longer it takes to publish a translation, we miss out in potential views in other languages, and we risk losing translators whose work is waiting for approvals," said Helena Batt, deputy director, TED Translators.
"Instead of starting subtitles from scratch, we tested machine transcriptions as a first step, before translators began their work. We measured time saved per talk, quality of machine translation, and accuracy. On average, volunteers subtitle talks 30% faster (when a video is machine-transcribed first), 75% agreed that machine translations were good quality. By pairing humans with AI, it's possible to translate much faster," said Jennifer Zurawell, director, TED Translators.
The goal: publishing 5 translations on the first day a video goes live.
_______________________________
1:44 PM
1:44 PM
Because of the translations, the TED brand is trusted as a relevant source of information around the world.
- 30k volunteers across 160 countries are critical to helping ideas spread freely.
- It takes 5-10 hours to accurately translate a TED talk, then review over several hours, before approval to go live.
- TED translators contributed 18k subtitles in 2019.
- Speaker style must be translated, not just the verbatim.
_______________________________
1:40 PM
1:40 PM
To make TED videos available globally, TEDTranslate crowdsources translations from volunteers, making TED Talks accessible in more than 100 different languages.
_______________________________
1:37 PM
1:37 PM
TED mobile app users:
5% Latin America
20% Europe
44% Asia
20% Europe
44% Asia
_______________________________
1:36 PM
1:36 PM
At a session titled "How TED uses AI to spread ideas farther and faster" - TED's mission is to "find the world's most inspired thinkers."
_______________________________
1:34 PM
1:34 PM
From re:MARS keynote with Robert Downey Jr.
01 / 05
_______________________________
1:17 PM
1:17 PM
From re:MARS keynote with Morgan Pope and Toni Dohi of Walt Disney Imagineers
Imagineers from Disney demo how figures are capable of untethered, dynamic movements -- rather than play back an animation, they can arbitrarily perform. Our motto at Walt Disney Imagineering Research and Development is fail fast and make better mistakes tomorrow.
At Disney, we're not in the business of making robots. We're in the business of making characters. So the challenge we worked through was to start to capture the moments of a performer to make you think, wow, this is a person.
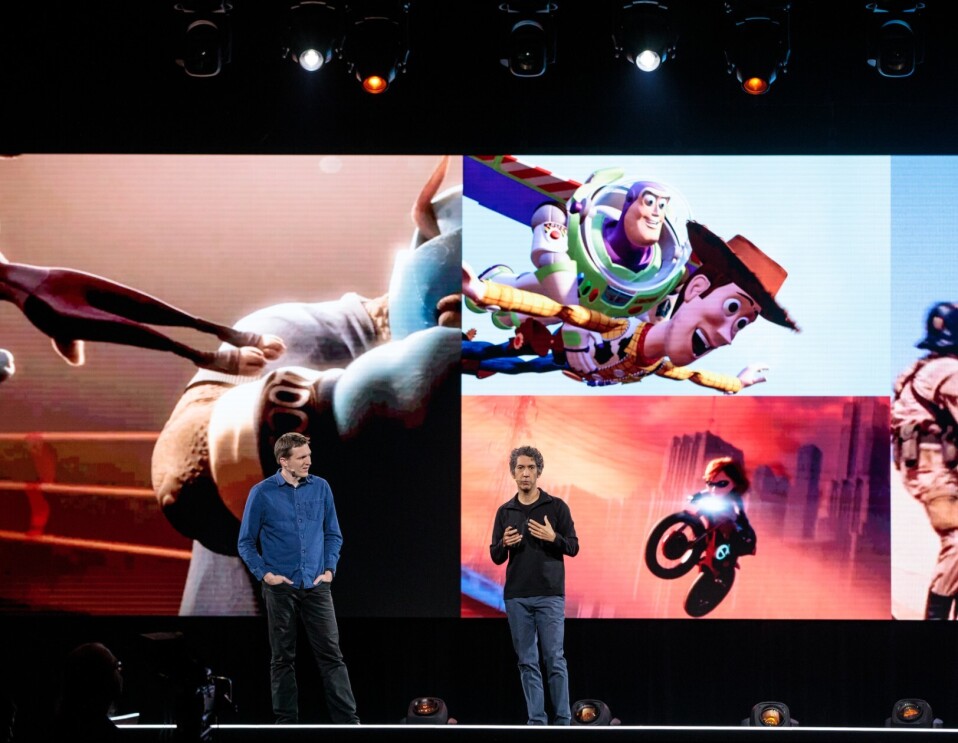
_______________________________
12:01 PM
12:01 PM
_______________________________
11:49 AM
11:49 AM
Now that the keynote and fireside chat have wrapped, we've got sessions to attend and share, then the party at the Las Vegas Speedway. Stay tuned for more photos, updates, and behind-the-scenes.
_______________________________
11:17 AM
11:17 AM
Jenny Freshwater: What advice would you give to anyone looking to start their own business?
Jeff Bezos: I get asked this question from time to time. The most important thing is to be customer-obsessed. Don't satisfy them, absolutely delight them.
Passion. You'll be competing against those who are passionate.
Take risk. You have to be willing to take risk, if you have a business idea with no risk, it's probably already being done. You've got to have something that might not work. It will be, in many ways, an experiment. We take risks all the time, we talk about failure. We need big failures in order to move the needle. if we don't, we're not swinging enough. You really should be swinging hard, and you will fail, but that's okay.
Everything I listed does not need to be at a startup. You could be at a fortune 500 company.
_______________________________
11:12 AM
11:12 AM
Jenny Freshwater: Why Blue Origin?
Jeff Bezos: We know so much more about the moon than we did before. (For example) it takes 24x less energy to lift a pound off the moon than the earth.
The moon is close to the earth, it's only 3 days away. It's logistically a very good place to go.
We need to move industry to the moon. Earth will be zoned residential.
You cannot start a space company from your dorm room - the infrastructure doesn't exist.
_______________________________
11:07 AM
11:07 AM
Jenny Freshwater: How do you deal with things that don't work?
Jeff Bezos: When do you know when to stop? When the last champion is ready to throw in the towel, it's time to stop.
_______________________________
11:06 AM
11:06 AM
Jenny Freshwater: What's something you're excited about?
Jeff Bezos: Project Kuiper -Satellites and broadband everywhere. When you put thousands of satellites around the world - you end up servicing the whole world.
_______________________________
11:03 AM
11:03 AM
Jenny Freshwater: Say you had a crystal ball, what is your 10 year prediction?
Jeff Bezos: I think you can foresee some things in the 10 year time-frame. I think grasping will be solved, Outside my arena of expertise, I think we'll see amazing advancements in biotechnology, we'll continue to see advances in machine learning and AI.
The bigger question is "what's not going to change in the next 10 years?" Look at what's stable in time, and continue to focus there. Identify those ideas, they're usually customer needs. If you're basing a strategy on customer needs that are stable in time (fast delivery, low cost), you can spin up a lot of flywheels around those questions. They're so big and fundamental.
_______________________________
10:59 AM
10:59 AM
Jenny Freshwater: Have you ever had trouble moving your ideas forward?
Jeff Bezos: We have a leadership principle, "disagree and commit," and a lot of times, I'll disagree and commit. Often, you're working with people who are just as passionate as you are, they have their own set of ideas. If you're the boss, the other people may have more access to the ground truth than you. Often the boss should be the one to disagree and commit.
_______________________________
10:56 AM
10:56 AM
Jenny Freshwater: If Amazon hadn't worked out, what would you be doing?
Jeff Bezos: If Amazon hadn't worked out, I'd probably have been an incredibly happy software engineer.
_______________________________
10:56 AM
10:56 AM
Jenny Freshwater: How do we " be right a lot?"
Jeff Bezos: If you say "be right a lot" that's not very helpful. People who are right a lot listen a lot, and they change their mind a lot. People who are right a lot change their mind without a lot of new data. They wake up and reanalyze things and change their mind. If you don't change your mind frequently, you're going to be wrong a lot. People who are right a lot want to disconfirm their fundamental biases.
_______________________________
10:53 AM
10:53 AM
Jenny Freshwater: Dave Limp told us about your library. Can you tell us about builders and dreamers?
Jeff Bezos: The dreamers come first, the builders get inspired by them. They build a new foundation so the dreamers can stand on it and dream more. Everyone is a builder and a dreamer. They stand on each other's shoulders.
_______________________________
10:51 AM
10:51 AM
Dr. Werner Vogels has returned to the stage, introducing Jenny Freshwater and Jeff Bezos to the stage.
_______________________________
10:48 AM
10:48 AM
"Bill Gates picked robot dexterity as the number one thing that will advance this year."
_______________________________
10:48 AM
10:48 AM
Working with a set of objects a robot has never seen before, a new robot has a gripper and suction cup, and it's able to pick up the objects very quickly.
This bot is able to pick up more than 400 objects per hour. if the bot fails to pick up an object, it must remember that it didn't work with that modality, and try the other one (gripper or suction cup) next time.
Some objects are a nightmare, for example a paper clip cannot be picked up by gripper or the suction cup.
_______________________________
10:43 AM
10:43 AM
He references an "arm farm" where dozens of robotic arms are picking up differently shaped and sized objects, 24/7 to collect data on grasping, lowering the failure rate to 20%.
_______________________________
10:40 AM
10:40 AM
He's talking about measuring the reliability and predictability of a grasp using Plato's theory.
_______________________________
10:37 AM
10:37 AM
"Put yourself in the position of being a robot. All of your sensors are noisy and imprecise, and your actuators driving your arm are imprecise. So it's not surprising that robots are clumsy."
_______________________________
10:35 AM
10:35 AM
Ken Goldberg, engineering professor, UC Berkeley and chief scientist, Ambidextrous Robotics has taken the stage.
_______________________________
10:32 AM
10:32 AM
"In 1971, President Nixon declared a war on cancer. War is the wrong metaphor - war is a state of fear and hate. Greed is sustainable. Why not put a price tag on the head of cancer and combine data science with financial engineering?"
_______________________________
10:28 AM
10:28 AM
"Missing data is the problem. Most studies do not report extent of missingness or use listwise deletion for medical trials."
_______________________________
10:26 AM
_______________________________
10:26 AM
"With modern AI, we can do better. We can apply data science techniques to medical trials."
_______________________________
10:23 AM
10:23 AM
"It's harder and harder to raise money for the early part of drug development. It takes $200 million to develop an anti-cancer drug over 10 years, with a 5% probability of success."
_______________________________
10:18 AM
10:18 AM
"The challenge is the cost of drug development. The smarter we get, the more complex is the science of medicine. If there's something investors do not like, it's complexity and risk."
_______________________________
10:16 AM
10:16 AM
"We are living in a biomedical inflection point in how we deal with illness."
_______________________________
10:13 AM
10:13 AM
Andrew Lo is now on stage.
_______________________________
10:12 AM
10:12 AM
"We are designing from the ground up for autonomy, rather than for human drivers."
_______________________________
10:11 AM
10:11 AM
"Instead of making millions of cars and serving millions of people, thousands of cars can serve millions of people."
_______________________________
10:09 AM
10:09 AM
"Our vehicle is designed to operate in a shared fleet. This is a much better use of resources."
_______________________________
10:07 AM
10:07 AM
"The human is never driving, they are giving permission."
"Each sensor on the vehicle can see 270 degrees, and we have multiple views. If one sensor fails, we still have a 360 degree view. We can see more, we process more, we can understand more. This is criticial for a vehicle designed for autonomy."
_______________________________
10:05 AM
10:05 AM
"We are not customizing only for San Francisco, the technology needs to be scalable."
_______________________________
10:04 AM
10:04 AM
"San Francisco is a complex place to drive. The variables in the city teach us to be even safer than humans."
_______________________________
10:03 AM
10:03 AM
"Basics of artificial intelligence are sensing, planning, and acting"
"The vehicle is computing it's preferred path, and predicts the behavior of other agents, for example, right of way of multiple agents at a 4-way stop, pedestrians who may change their mind, a double parked car. It can adjust it's path in real-time."
_______________________________
9:59 AM
9:59 AM
"We spend 400 billion hours driving each year. We only use our cars 4% of the time. 1/3 of the traffic in cities is actually people searching for parking. 1/4 of air pollution is caused by transportation. Car crashes take the lives of 1.4 million people each year. Over 90% of those crashes are caused by human error."
_______________________________
9:55 AM
9:55 AM
_______________________________
9:55 AM
9:55 AM
"In eras of technological disruption, leadership matters. We must invest in education, informed government leaders, to take everyone along with us."
_______________________________
9:51 AM
9:51 AM
"We need to move from big data to small data. Much of AI has been led by software companies, but many smaller companies do not have the luxury of all that data. Could 100 examples be enough to support machine learning?"
_______________________________
9:46 AM
_______________________________
9:46 AM
"Often the #1 project the CEO recommends is not the one to invest in."
(laughter)
_______________________________
9:45 AM
(laughter)
_______________________________
9:45 AM
"Executives #1 problem is how to find the right use cases."
Here's how to start:
- Start small - I've seen more teams fail by going big, than by going small (aim for a 4-6 month project)
- Automate tasks, not jobs - As a framework for brainstorming AI ideas, look at a job, then break down the tasks, and identify the ones that could have AI enter the task
- Combine AI and subject matter expertise - Chose projects at the intersection of what AI can do and things that are valuable for your company (cross functional teams can help prioritize)
_______________________________
9:40 AM
9:40 AM
"The sheer volume of ideas are out there on the internet for anyone to use (referencing academic papers). AI papers are being published at a rate of 100 per day."
_______________________________
9:38 AM
_______________________________
9:38 AM
"The rise of talent, ideas, and tools is making AI more accessible than ever before, even to companies outside of tech."
_______________________________
9:36 AM
_______________________________
9:36 AM
"This is the time to bet on AI outside of the tech industry."
_______________________________
9:35 AM
_______________________________
9:35 AM
"AI is the new electricity."
_______________________________
9:35 AM
_______________________________
9:35 AM
"We've created the world's first global autonomous racing league with Deep Racer, putting machine learning into the hands of every developer."
_______________________________
9:31 AM
_______________________________
9:31 AM
"Every engineer can become a machine learning engineer. We've launched Amazon Machine Learning Academy, which is what we use to train our engineers, and it's available right now."
_______________________________
9:29 AM
_______________________________
9:29 AM
"Netflix says 75% of their watching is by recommendations, so your recommendations better be good."
_______________________________
9:27 AM
_______________________________
9:27 AM
"You don't need to build recommendation models, we've already done that for you."
_______________________________
9:26 AM
_______________________________
9:26 AM
"The quality of your data is increasingly important."
_______________________________
9:24 AM
_______________________________
9:24 AM
Werner Vogels shares how NFL is able to share real-time data thanks to sensors and machine learning.
_______________________________
9:23 AM
_______________________________
9:23 AM
"We have quite a few customers who are building their system using Sagemaker. For example, Intuit built an expense finder tool that looks at a year of financial data to identify deductible expenses. In the past, that kind of work would have taken 6 months to build a new model. With Sagemaker, it takes just one week."
_______________________________
9:22 AM
_______________________________
9:22 AM
"We started offering machine learning as a service, like Sagemaker, now every single developer is a machine learning developer."
_______________________________
9:17 AM
_______________________________
9:17 AM
"85% of cloud computing happens on AWS."
_______________________________
9:17 AM
_______________________________
9:17 AM
"To best support customers with machine learning, we needed to build a completely new stack."
_______________________________
9:13 AM
_______________________________
9:13 AM
"We're taking machine learning out of the hands of data scientists, and into the hands of developers. We're democratizing machine learning."
_______________________________
9:11 AM
_______________________________
9:11 AM
"Companies of every size and industry are making use of machine learning."
_______________________________
9:07 AM
_______________________________
9:07 AM
"AWS now has 165 services for you, whether you want to do analytics, remote desktop, mobile services, devops, running ML models, etc."
_______________________________
9:05 AM
_______________________________
9:05 AM
"We integrate security into all that we do, including machine learning."
_______________________________
9:05 AM
_______________________________
9:05 AM
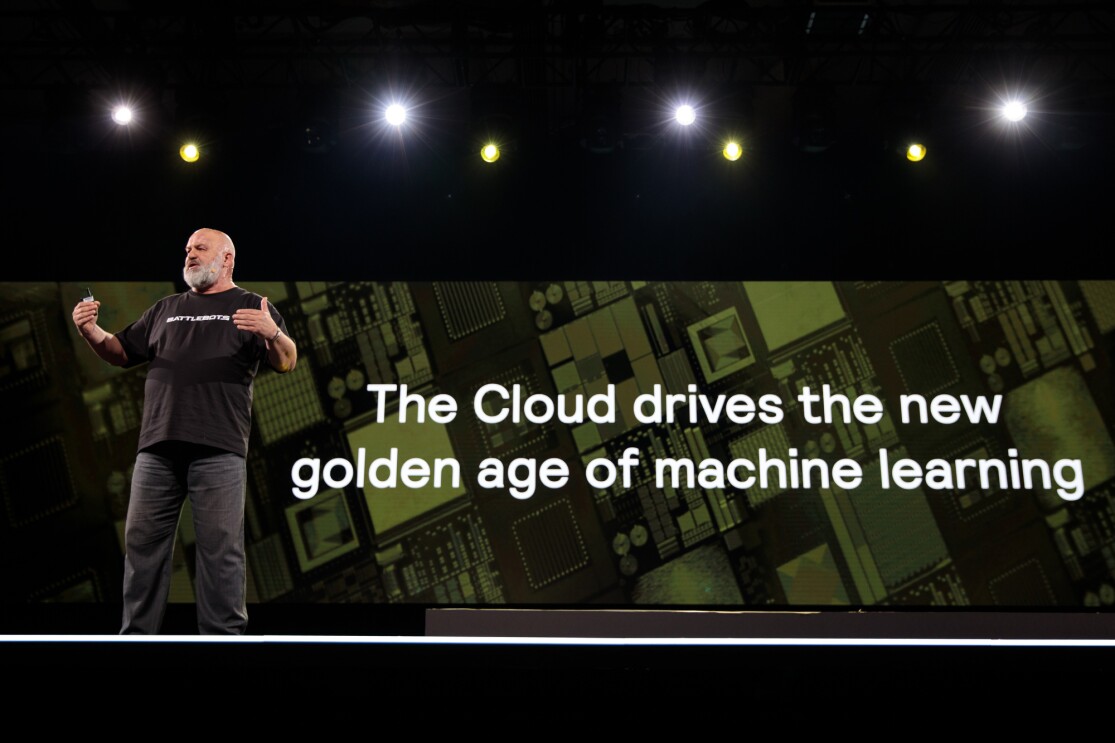
"AWS has driven so much innovation around the world. Many successful companies say they wouldn't have existed without cloud computing."
_______________________________
9:02 AM
_______________________________
9:02 AM
"We've been doing machine learning at Amazon for the past 25 years."
"Amazon has been the pioneer in cloud computing. We launched our first services in 2006. We built these technologies for ourselves."
_______________________________
10:05 AM
_______________________________
10:05 AM
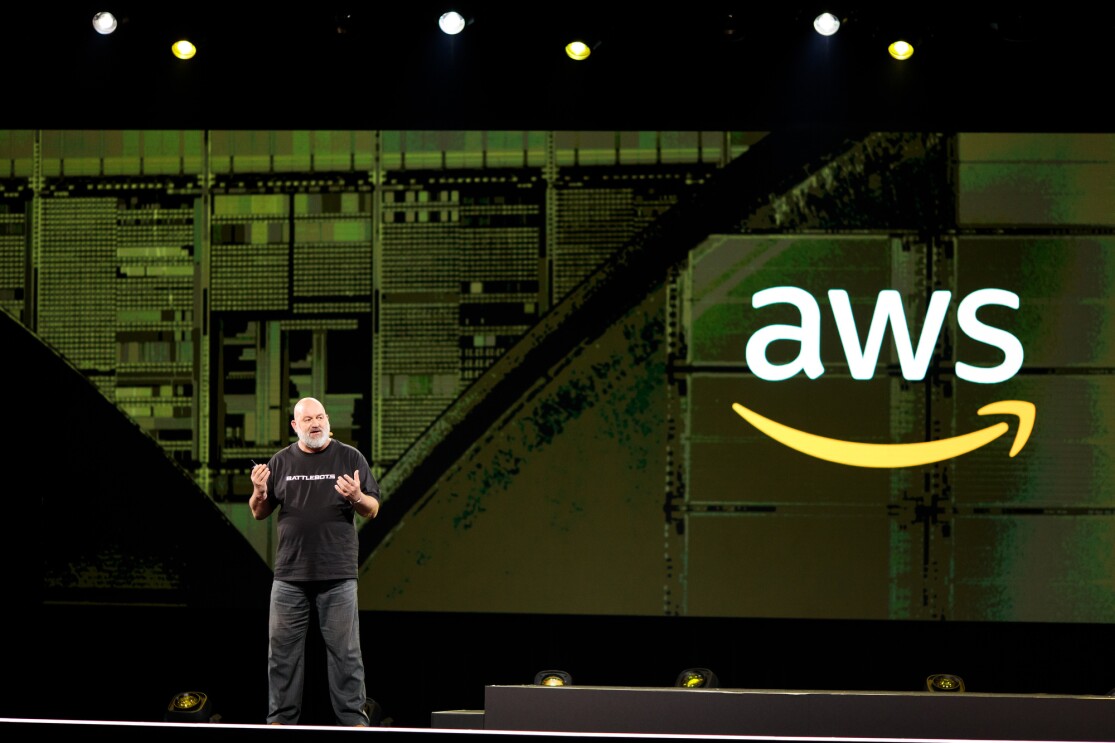
The keynote is beginning, with Dr. Werner Vogels taking the stage.
_______________________________
8:44 AM
_______________________________
8:44 AM
_______________________________
8:01 AM
8:01 AM
Last night, Jeff Bezos met with Leo, one of the first Amazon Future Engineer scholarship recipients at re:MARS.
_______________________________
7:50 AM
06/06/19
7:50 AM
06/06/19
We're back with our third day at re:MARS! We'll start with a keynote featuring Dr. Werner Vogels, CTO of Amazon, Andrew NG, Founder and CEO of Landing AI and deeplearning.ai, Aicha Evans, CEO of Zoox, Andrew Lo, The Charles E. and Susan T. Harris Professor at MIT Sloan School of Management and director of MIT Laboratory for Financial Engineering, and Ken Goldberg, engineering professor at UC Berkeley and chief scientist at Ambidextrous Robotics. Then our fireside chat, breakout sessions, and, of course, the party!
_______________________________
9:27 PM
_______________________________
9:27 PM
This day has been filled with robots, drones, and lots of learning.
We'll be back in the morning with another keynote, a fireside chat with Jeff Bezos and Jenny Freshwater, and more sessions, wrapping up with an event at the Las Vegas Speedway.
_______________________________
9:27 PM
_______________________________
9:27 PM
_______________________________
8:26 PM
8:26 PM
_______________________________
5:55 PM
5:55 PM
Jeff Wilke, CEO consumer worldwide, continues the Wednesday morning re:MARS keynote, speaking about how Amazon has been exploring drone delivery.
_______________________________
5:38 PM
5:38 PM
Jeff Wilke opened this morning's keynote before welcoming other Amazon leaders to the stage. Watch for details on what he shared.
_______________________________
4:32 PM
4:32 PM
From re:MARS session "Enabling sustainable human life in space using artificial intelligence" by Natalie Rens, CEO and founder, Astreia and Koorosh Lohrasbi, solutions architect, Amazon Web Services
Details:
One of the most challenging aspects of being on Mars is the isolation. This is the view from Mars.
One of the most challenging aspects of being on Mars is the isolation. This is the view from Mars.
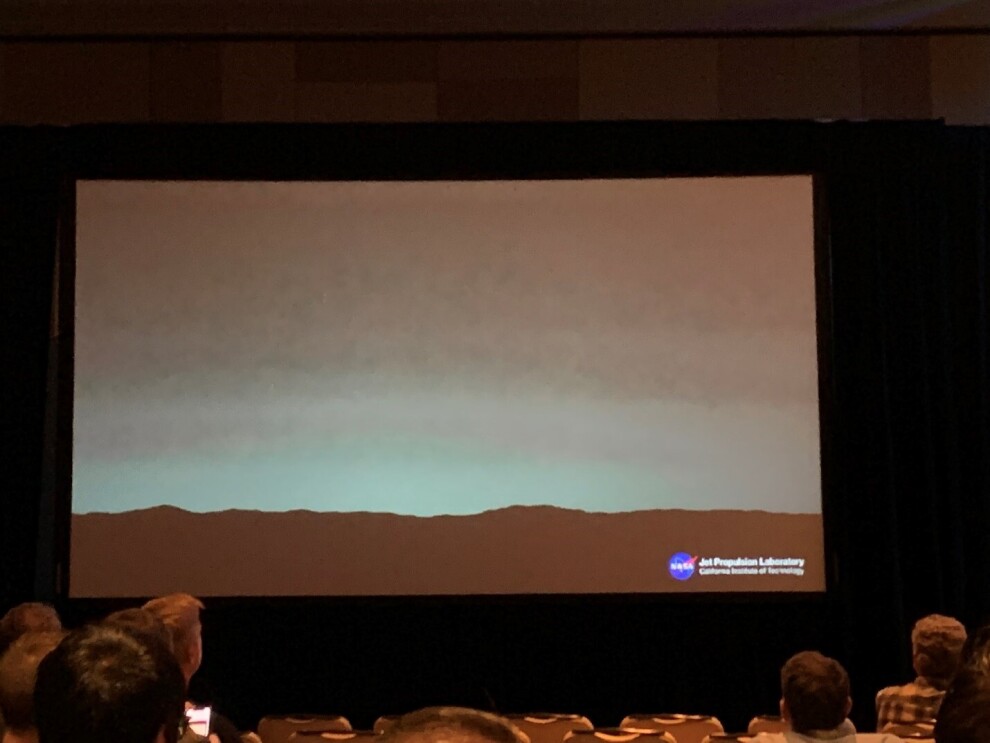
If something were to go wrong on Mars, a communication sent at the speed of light at the current orbital positions would take 20 minutes to get to Earth. We can’t operate the way we do today. Artificial intelligence (AI) becomes a must.
Digital habitats will have to mirror our physical worlds, and will become essential to being able to sustaining life on Mars.
_______________________________
4:14 PM
4:14 PM
Leadership on stage during re:MARS Wednesday keynote.
Hear from
- Dilip Kumar, VP Amazon Go
- Jenny Freshwater, director, forecasting
- Brad Porter, VP & distinguished engineer, robotics
- Rohit Prasad, VP & head scientist, Alexa
_______________________________
3:49 PM
3:49 PM
CYMI: Marc Raibert's keynote presentation at re:MARS first night. He spoke about Boston Dynamics robots, and demoed two robot dogs.
_______________________________
2:48 PM
2:48 PM
Another robot we saw at the Tech Showcase, this bot works to minimize repetitive (but important) tasks in a process.
_______________________________
2:41 PM
2:41 PM
Seen at the Tech Showcase, this robot sorts items by color and places the items in a jar. We got to race against the bots, and (no surprise) we didn't win.
_______________________________
12:55 PM
12:55 PM
In the Tech Showcase, screens on robotic arms greet attendees.
_______________________________
11:29 AM
11:29 AM
Keynote is ending. We'll be moving on to details from workshops and sessions, shortly.
_______________________________
11:20 AM
_______________________________
11:20 AM
"iRobots vacuums were focused on solving a problem.
The promise is that you put them in your home and you never have to vacuum again. But they would get caught in fringe carpet. You had to push an "on" button.
We built it in a way that it would see the dirt and be smart enough to do the job. They added a feature to the Roomba (a homebase) that would empty the Roomba once it was done, so customers could go a year or two without emptying the Roomba. Each advancement is making the robot more autonomous."
_______________________________
11:13 AM
11:13 AM
Colin Angle, CEO and founder of iRobot is now on stage.
_______________________________
11:00 AM
_______________________________
11:00 AM
_______________________________
10:58 AM
10:58 AM
Kate Darling, research specialist at the MIT Media Lab and affiliate at the Harvard Berkman Center is now on stage.
She's speaking about robotics and how people consciously or subconsciously treat robots like living things. For example, more than 80% of Roombas have a name.
_______________________________
10:43 AM
_______________________________
10:43 AM
Daphne Koller, CEO and founder of Insitro has taken the stage.
"In the last ten years ML has made incredible progress."
In 2019, computers are able to label photographs with natural language, but as recent as 2005, they may've not been able to share more than a yes/no response about a specific object in an image.
_______________________________
10:32 AM
_______________________________
10:32 AM
He's speaking about new satellite technology, Mars rovers, including a Mars helicopter rover coming in 2020.
How do we democratize a Mars rover? Get involved.
_______________________________
10:21 AM
10:21 AM
Automated intelligence is always at our fingertips.
How do we make IT limitless?
Leverage IoT, programming, analytics, smart data, cloud and AI which can evolve at different cadences. Leverage data from the clouds via APIs, so we can make much better decisions faster. Apply AI and ML to make it better and faster all the time.
_______________________________
10:17 AM
_______________________________
10:17 AM
Tom Soderstrom, IT chief technology and innovation officer at Jet Propulsion Laboratory takes the stage.
_______________________________
10:12 AM
_______________________________
10:12 AM
The tech industry is abuzz with all-things-drones. But not all drones are created equal. Some are remotely-piloted drones. Some drones are autonomous but rely on ground-based communications systems for situational awareness, meaning they aren’t able to react to the unexpected. Then there are systems like Amazon’s — independently safe.
Using a sophisticated, industry-leading sense-and-avoid system helps ensure our drones operate safely and autonomously. If the environment changes, and the drone‘s mission commands it to come into contact with an object that wasn’t there previously – it will refuse. Building to this level of safety isn’t an easy task. But from the start, the choice was clear. In order for this to make a difference at Amazon’s scale, a safe, truly autonomous drone was the only option for us.
Before I finish up, I wanted to leave you all with a little glimpse of Amazon’s future. This is our latest drone.
_______________________________
10:05 AM
10:05 AM
Last year, we launched Amazon Future Engineer, a program designed to ensure all students – especially students from underrepresented communities – have access to computer science education so that someday, they might choose to pursue a career in AI.
We have an ambitious goal: to inspire more than 10 million kids each year to explore computer science through coding camps and online lessons. We’ll fund introductory and Advanced Placement courses in computer science for more than 100,000 young people in 2,000 low-income high schools across the U.S. This spring, we awarded 100 students pursuing degrees in computer science with four-year, $10,000 annual scholarships. Some of those students have already started their Amazon internships.
Leo Jean Baptiste, one of the 100 students who received the inaugural Amazon Future Engineer scholarship, has joined us here today.
_______________________________
10:03 AM
10:03 AM
Some ways we've balanced the benefits of artificial intelligence with any potential risks:
Our partnership with the National Science Foundation, committing up to $10 million in each of the next three years to research grants focused on fairness in AI. And we have joined with companies and organizations such as Microsoft, Apple, The Allen Institute for AI, OpenAI and others to form the Partnership on AI. We work with academia and government organizations like the National Institute of Standards and Technology to ensure our technology can be tested and evaluated for fairness and accuracy.
_______________________________
10:00 AM
Our partnership with the National Science Foundation, committing up to $10 million in each of the next three years to research grants focused on fairness in AI. And we have joined with companies and organizations such as Microsoft, Apple, The Allen Institute for AI, OpenAI and others to form the Partnership on AI. We work with academia and government organizations like the National Institute of Standards and Technology to ensure our technology can be tested and evaluated for fairness and accuracy.
_______________________________
10:00 AM
Behind all of this invention is the computing and AI horsepower provided by Amazon Web Services.
AWS sees the importance of making AI accessible to everyone. Not just for data scientists, but also for developers and anyone who wants access to the technology. So on top of the value of the cloud – which supports enormous volumes of data and processing power – AWS has built the broadest selection of tools for customers to use, regardless of how new or how sophisticated they are with their use of AI.
We want to enable enterprises and companies to be able to use machine learning in an expansive way, and that’s why AWS launched services like Amazon SageMaker, a managed ML service that takes a number of the required steps like:
- Collect and prepare training data
- Choose and optimize your ML algorithm
- Set up and manage environments for training
- Train and tune the model
- Deploy ML to production
- Scale and manage the production environment
… and makes the process much more addressable by everyday developers and scientists.
- Collect and prepare training data
- Choose and optimize your ML algorithm
- Set up and manage environments for training
- Train and tune the model
- Deploy ML to production
- Scale and manage the production environment
… and makes the process much more addressable by everyday developers and scientists.
We have AI services that loosely mimic human cognition and enable developers to plug-in pre-built AI functionality into their apps without having to worry about the machine learning models that power these services.
_______________________________
9:56 AM
_______________________________
9:56 AM
This isn’t just a vision. I’m excited to announce that we will be rolling out this night out experience soon to customers. I want to thank our early developers for going on this journey with us and look forward to working together in bringing many such magical experiences to our customers.
_______________________________
9:56 AM
_______________________________
9:56 AM
Each skill that is built through Alexa Conversations capability has a deep recurrent neural network to predict different dialog actions within a skill. We now have another recurrent neural network that acts as a cross-skill predictor. At any given turn, this cross-skill action predictor determines whether it should hand off the dialogue control to another skill or keep the control with the current skill. The cross-skill action predictor is also trained on simulated dialogues just like within-skill dialogue action predictor.
_______________________________
9:52 AM
_______________________________
9:52 AM
This development allows for experiences such as ‘Alexa, what movies are playing nearby?’, and Alexa then stitches together the capabilities of multiple providers at once – in this case movie recommendation and ticket booking; restaurant recommendation and reservation; and a rideshare.
The approach is different from other dialogue systems in that it models the entire system end-to-end: the system takes spoken text as its input, and delivers actions as its output.
We had to overcome a significant scientific challenges with this approach: modeling a massive system state space of a complex dialogue experience – the set of actions to take given all possible permutations of entity values, context, and dialogue state.
We solved this by enabling the runtime system to directly predict the next action, which can be either a service-specific API call or an Alexa response. The action prediction is based on a machine-learned conversational model that takes the entire dialogue history into account.
_______________________________
9:51 AM
_______________________________
9:51 AM
Now, we have advanced our machine learning capabilities such that Alexa can predict customer’s true goal from the direction of the dialogue, and proactively enable the conversation flow across skills
_______________________________
9:51 AM
_______________________________
9:51 AM
Automated dialog flow within a skill is a big leap for conversational AI. But customers don’t limit their conversations to a single topic or a single skill. Imagine a customer that begins a conversation by asking Alexa about movie showtimes and tickets, but her true intent is to plan a night out with her family. With today’s AI assistants, she would reach her goal by organizing the tasks across independent, discrete skills. In such a setting all the cognitive burden is with the customer.
_______________________________
9:50 AM
_______________________________
9:50 AM
Today, I am excited to announce the private preview of Alexa Conversations, a deep learning-based approach for creating natural voice experiences on Alexa with less effort, fewer lines of code, and less training data than ever before. Hand coding of the dialog flow is replaced by a recurrent neural network that automatically models the dialog flow from developer provided input. With Alexa Conversations, it’s easier for developers to construct dialogue flows for their skills.
_______________________________
9:49 AM
_______________________________
9:49 AM
To provide more utility we envision a world where customers will converse naturally with Alexa: seamlessly transitioning between topics, asking questions, making choices, and speaking the same way that you would with a friend, or family member.
_______________________________
9:48 AM
_______________________________
9:48 AM
We are focused on AI advances that translate to better customer experiences across 4 pillars.
Trust: Customer trust is paramount to us. Our guiding tenet is transparency and providing controls to customers on information they share with Alexa.
Smarter via Self-Learning: To reduce our reliance on human labeling and enable Alexa to learn faster, we are continually inventing on semi-supervised and unsupervised learning capabilities.
Proactive: We’ve made Alexa more proactive and helpful by incorporating context of Who, What, When, and Where.
Natural: The key to making Alexa useful for our customers is to make it more natural to discover and use her functionality.
_______________________________
9:47 AM
Natural: The key to making Alexa useful for our customers is to make it more natural to discover and use her functionality.
_______________________________
9:47 AM
Through several advances in machine learning, we have made Alexa 20% more accurate in the last 12 months.
_______________________________
9:46 AM
_______________________________
9:46 AM
Our inspiration for Alexa was the Star Trek computer – where customers could interact with a wide range of services and devices by just voice. To bring this “ambient computing” vision to fruition, we had to invent novel techniques for far-field speech recognition, natural language understanding, and text-to-speech synthesis.
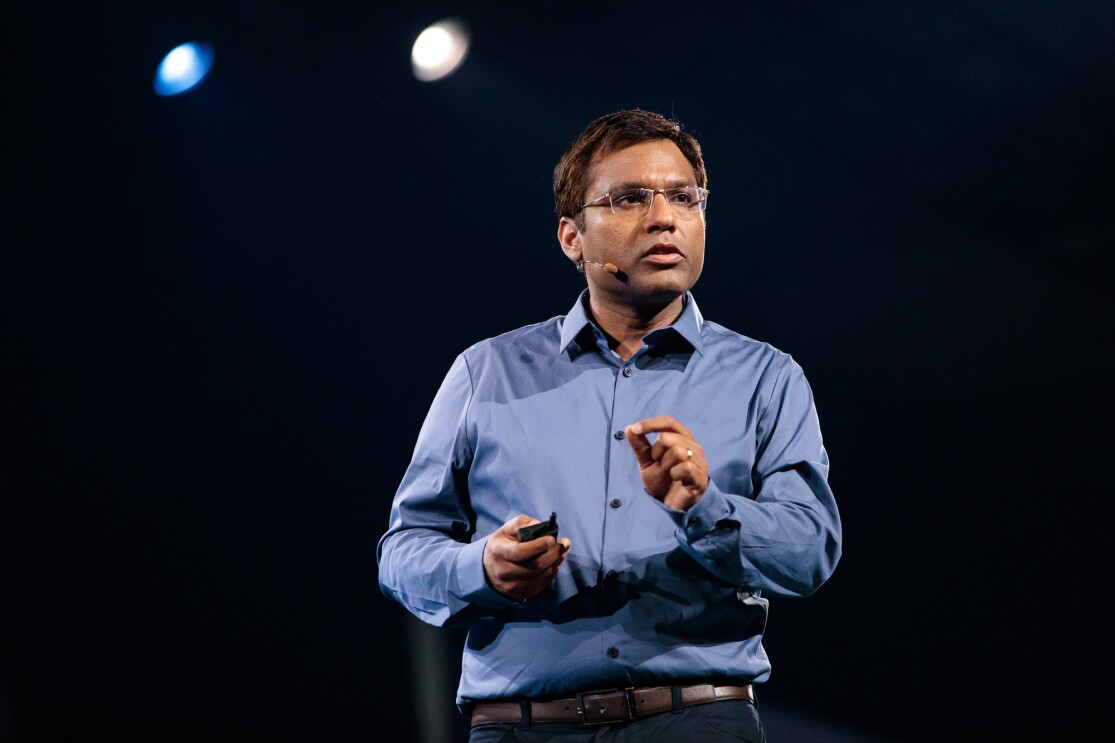
_______________________________
9:45 AM
9:45 AM
Next up is Rohit Prasad, VP and head scientist of Alexa.
_______________________________
9:43 AM
_______________________________
9:43 AM
We will also be able to combine this drive with the autonomous mobility
This will give us the ability to create fully autonomous drives that can collaborate with humans outside the robotic field. These innovations will enable us to bring more robotic automation to our processes even faster.
But we didn’t finish there. With the launch of the Pegasus sortation system, we realized we had the opportunity to rethink our drive design entirely. Let me show you for the first time, out new Xanthus drive, the base of our brand new Xanthus family.
The first vehicle in this family is our X-Sort Drive.
_______________________________
9:41 AM
_______________________________
9:41 AM
And it just works better, this technology can reduce the number of miss sorts by 50% over our other sortation solutions.
_______________________________
9:39 AM
_______________________________
9:39 AM
We’re excited today to share with you our new Pegasus Drive Sortation solution. The Pegasus solution leverages the core technology of our Amazon Robotics storage floors to transport individual packages to one of hundreds of destinations.
Our Pegasus drives receive individual packages onto their tabletop conveyor, travel across the elevated mezzanine, and ejects the package into the desired chute associated with the destination sort point.
_______________________________
9:37 AM
9:37 AM
We've also added more than just drive units to our buildings. Our robotic palletizers have already stacked more than two billion totes.
And safety is foremost in our robotic designs and our new robotic tech vests allow associates to walk onto the robotic fields confident the drives will stay far away.
But we can't stop there. With new benefits such as Prime One Day, speed is paramount and we recognized we needed another step function change in robotics… but this time in our middle-mile logistics.
_______________________________
9:36 AM
_______________________________
9:36 AM
The heart of our operations is what we like to call “the symphony of humans and robots” working together to fulfill and deliver customer orders. our robotics program started with Amazon's acquisition of Kiva Systems in 2012.
Since that acquistion seven years ago, we have deployed over 200,000 robotic drives, making jobs both easier and safer., while also increasing building storage capacity by 40%.
While these robots provide a critical function in our buildings, we are not automating away all the work. In that same timeframe, we have added over 300,000 full time jobs around the world.
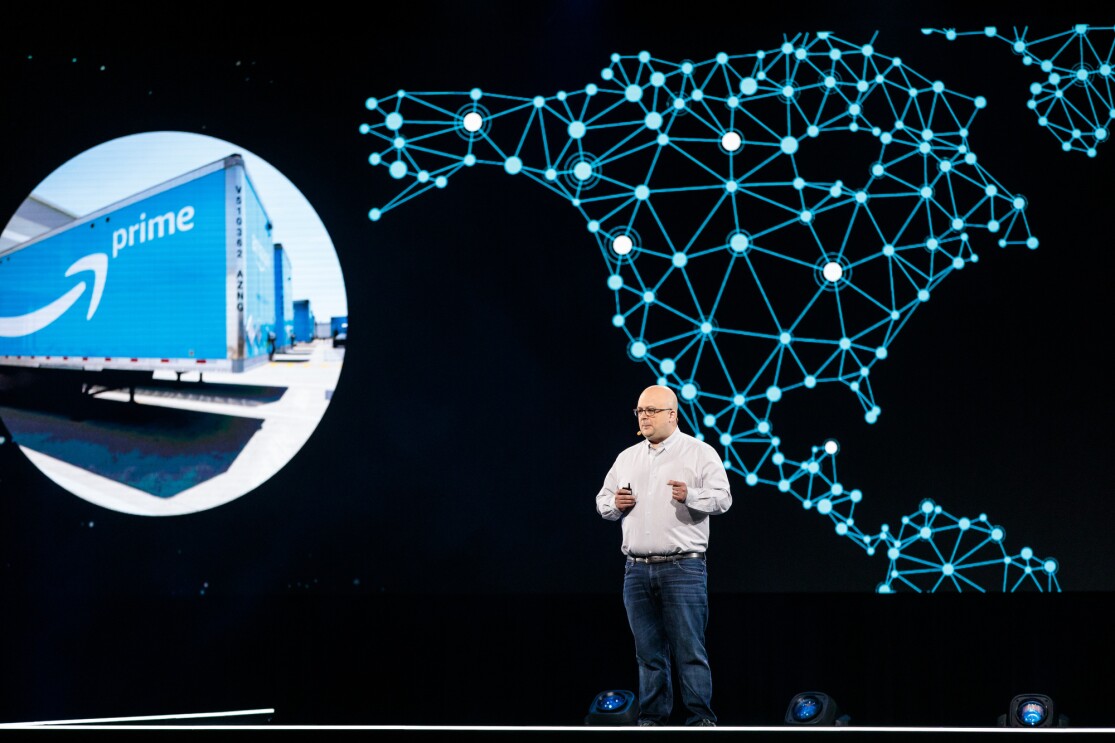
_______________________________
9:36 AM
9:36 AM
Brad Porter, VP and distinguished engineer of robotics is taking the stage.
_______________________________
9:35 AM
_______________________________
9:35 AM
A video is displayed during the keynote, showing how AI helps Amazon deliver.
_______________________________
9:33 AM
9:33 AM
What does it all mean?
If we have 400 million items for which we need to forecast demand, it’s clearly impossible for a person or team of people to create unique forecasts for those items. These neural networks allow us to build individual forecasts for every country in the world where Amazon operates, with potentially varying demand patterns for the same product by country without having human input for each item or even groups of items.
Take a niche product (a flip sequin pillow with Nicolas Cage), for example. We wouldn’t spend time forecasting demand for this item. But a neural network that is trained with the appropriate loss function can access 1000’s of similar products past histories of demand to give us an accurate enough idea of demand for the obscure product, and a high probability that when a customer in Las Vegas clicks to purchase the item, we can have it on her doorstep within two days.
_______________________________
9:30 AM
_______________________________
9:30 AM
These newer models are built around the state-of-the-art Convolutional Neural Networks (CNN) with automated feature engineering. They directly access historical demand needed for prediction. The generality of the CNN framework also allows us to borrow statistical strengths from the massive data across domains. The model can use the common dynamics learned from long-history products to help forecast new products, and vice versa.
For example, when a new version of a digital camera is introduced to the market, the models use the data from the previous version to predict future demand for the new camera.
And, while we are building models that are automated and learn on their own, our team has still tripled in size.
_______________________________
9:29 AM
_______________________________
9:29 AM
In 2016, we built a new Feed Forward Neural Network (FNN) model.
We were able to predict distributions for hundreds of millions of products by outputting quantiles for multiple forecast start dates and planning period combinations for up to one year ahead.
While the FNN architecture was successful, it required substantial efforts in time series feature engineering. In other words, months of manual training for each model and country we launched. That manual process meant slower model building and deployment for new use cases. To address these issues, we developed alternative neural network architectures.
_______________________________
9:28 AM
_______________________________
9:28 AM
Deep Learning models, (Neural Networks) have been around since the 1980s.
Recent computational breakthroughs with Deep Learning have led to real-world successes, and now this sub-field of machine learning is widely used for predictive modeling and the processing of images, text, and speech.
Amazon began deploying Deep Learning models to forecast item demand in 2015. Each year, we measure the success of our forecasting algorithms through various accuracy measurement. Prior to the use of deep learning as part of our forecasting system, we would achieve small incremental improvements each year. The year we launched deep learning, we achieved accuracy improvements up to 15x of what we had achieved in previous years. Improved forecasting accuracy translates directly to higher availability of the products our customers want, faster shipping speeds and lower costs.
_______________________________
9:28 AM
_______________________________
9:28 AM
Developed our first ML model, Sparse Quantile Random Forrest or SQRF for short, which represented a significant technical innovation for Forecasting in machine learning.
Was based on the popular Random Forest algorithm which enables decision trees. We implemented this algorithm from scratch using map-reduce, making it scale to billions of training examples.
This innovation in handling sparse numerical, categorical and text-based features with missing input values allowed our forecasting system to consume information from such as product description and title, in addition to demand time series.
It was also able to generate a full probability distribution of forecasts directly represented as samples of demand realizations for each time point in the forecast horizon.
While SQRF was useful in exploiting similarities across training samples using attributes, these decision tree models are not able to ‘borrow strength’ from samples with time series data.
_______________________________
9:27 AM
_______________________________
9:27 AM
We began adding machine learning methods to our forecasting back in 2007 to tackle two of the most difficult problems we face – predicting demand for new products and estimating the correct demand for products that are highly seasonal, such as Halloween costumes.
They’re seasonal, and you never know which costumes will see demand spikes.
_______________________________
9:27 AM
_______________________________
9:27 AM
It would be relatively easy to promise two-day delivery on products whose demand is predictable or linear.
Laundry soap, crackers, trash bags. And seasonal items – wool socks, sunscreen – have predictable enough demand patterns to put those items in the right fulfillment center in the right amounts, close enough to customers to make two-day shipping a reasonable promise.
No other company comes close to attempting the feats of logistical complexity we take on daily at Amazon. Consider some of the variables involved in whether we stock an item and where to have an item in inventory:
- We need to account for price elasticity and adjust for demand spikes when prices fall, for example like TVs.
- We need to understand how to differentiate between a slow-moving product and a product that won’t sell at all – like pickle-flavored lip balm which, believe it or not, isn’t really a top seller…
- And we need to predict regional demand so that we can distribute national forecasts across 10,000 zip codes and about a dozen shipping options.
_______________________________
9:26 AM
9:26 AM
The complexity of managing that scale is mind-bending, especially when you consider that some of those suppliers are large manufacturers and some are garage startups. And that a mom-and-pop seller has come to expect its products will get the same treatment as products from some of the largest brands in the world. The customer, meanwhile, often doesn’t know – or want to know – which product comes from which supplier. This becomes increasingly complex when we have to deliver items in two days or less with Prime, or an hour or less in cities where we operate Prime Now.
_______________________________
9:25 AM
_______________________________
9:25 AM
When you think about Artificial Intelligence and a supply chain, you might be tempted to think about robots and test runs with automated trucks. But AI’s impact on Amazon’s customers is far broader than the hardware side of the equation. Think about forecasting demand in the context of Amazon, a global company that ships billions of packages a year, has hundreds of millions of products to choose from and millions of suppliers, across every country that we serve.
_______________________________
9:23 AM
_______________________________
9:23 AM
Moving to the next topic, Jenny Freshwater is about to talk about how we use AI to power forecasting, the foundation of Amazon’s supply chain.
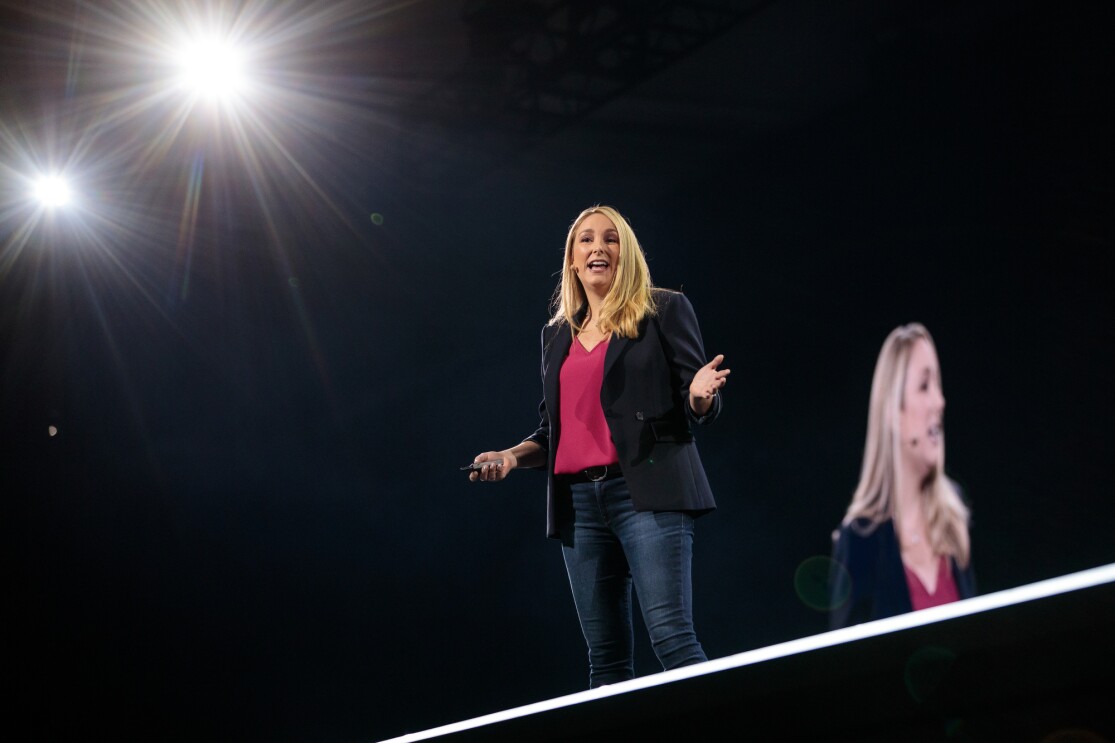
_______________________________
9:22 AM
9:22 AM
But that wasn’t the hardest part of it all – ensuring that the technology receded into the background of the store so customers had a seamless and magical experience was the hardest part. Customers just use an app to enter, shop like normal, and get a receipt after they leave the store.
It should be seamless and magical.
_______________________________
9:22 AM
_______________________________
9:22 AM
Product identification is another challenge as products look different under various circumstances or have very similar variants.
Machine learning algorithms are designed to improve with more data, but when you have a highly accurate system, there are few errors or negative examples to train the algorithms. So the team built synthetic data to train our algorithms, but had to ensure that no artifacts from the synthetic data incorrectly trained our deep networks.
The custom-built algorithms don’t get to showcase themselves without the right infrastructure that the team also built. We tackled many problems that are hard to solve but essential to hit our high accuracy bar for our customer experience.
_______________________________
9:18 AM
_______________________________
9:18 AM
There were several technology challenges the team had to overcome. First, determining customer account location to generate accurate receipts. Easy to do when there are just a few people in the store, but stores are much busier.
Dilip showed the audience a clip of the anonymized 3-D point clouds representing the output of the computer vision algorithms predicting customer account location in the store. The team had to build several algorithms that utilized geometry and deep learning to accurately predict customer account location and accurately associate interactions to the right customer account with very low latency.
_______________________________
9:17 AM
9:17 AM
Amazon Go’s technology is aiming to determine “who took what?” so we can accurately charge customer accounts for taken products.
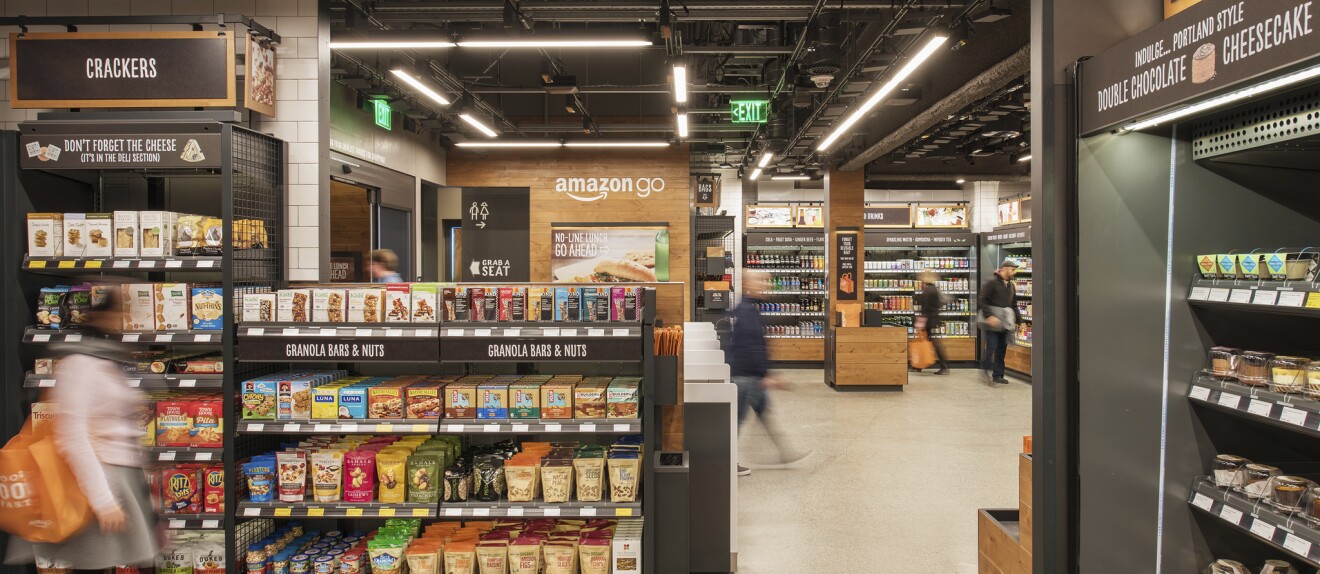
_______________________________
9:17 AM
9:17 AM
We considered lots of different technology options, but had a strong hypothesis that computer vision would provide the perfect palette to create the seamless Amazon Go customer experience.
_______________________________
9:16 AM
_______________________________
9:16 AM
Today there are 12 Amazon Go stores across Seattle, Chicago, San Francisco, and New York, all utilizing our Just Walk Out Technology.
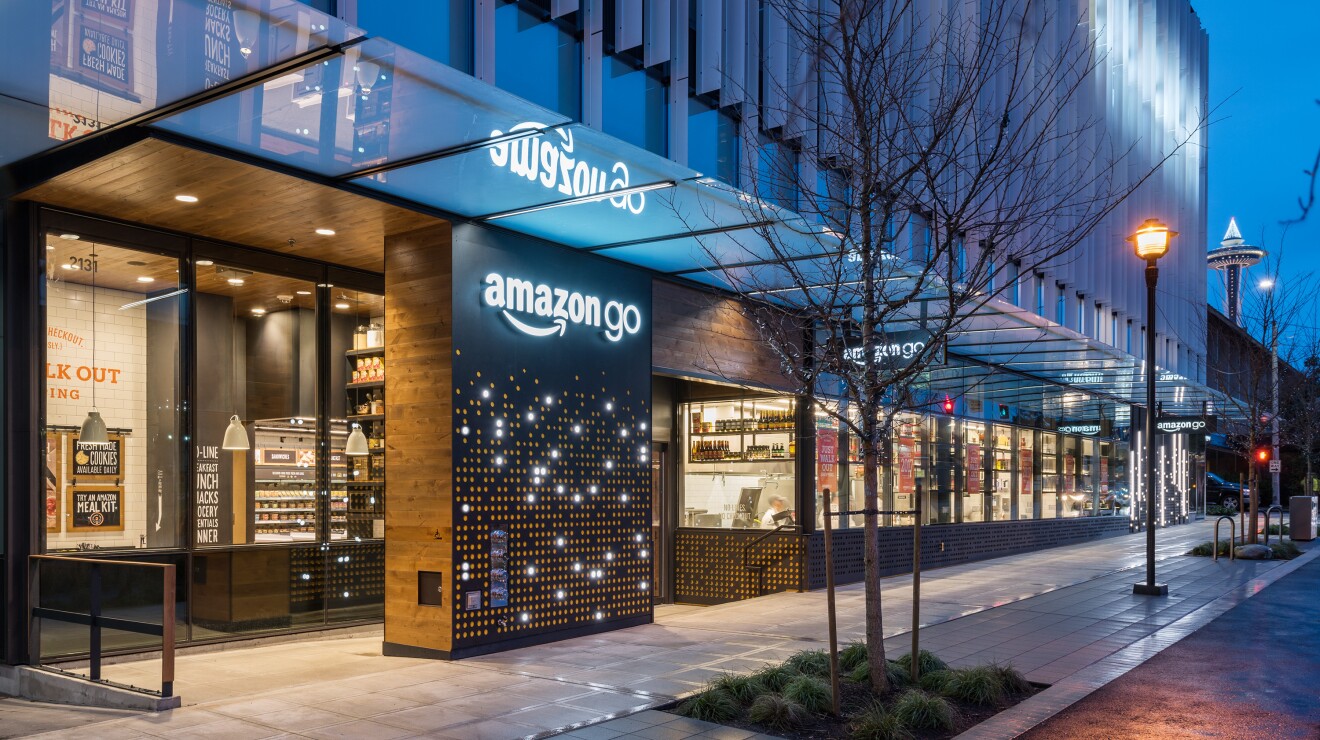
_______________________________
9:16 AM
9:16 AM
Amazon Go is a checkout-free store that enables customers to take what they want, then just walk out.
How did the idea originate? Several years ago, a small group formed to determine how to make the physical retail experience even better. They realized that no one likes to stand in line, so we set out to create an experience where customers could come in, take what they want, and just walk out.
_______________________________
9:15 AM
_______________________________
9:15 AM
Dilip Kumar, Vice President of Amazon Go, takes the stage to detail the innovative Just Walk Out Technology behind Amazon Go.
_______________________________
9:15 AM
_______________________________
9:15 AM
StyleSnap uses image recognition and deep learning to i.d. an apparel item and recommend similar items, deep learning supports object detection to identify the various apparel items in the image and categorize them into classes, such as fit-and-flair dresses or flannel shirts. We use a deep embedding model to define visual similarity, and the model even ignores the differences between catalog and lifestyle images and instead focusus on the unique color, pattern, and style elements that customers are looking for. Get a deeper dive into how StyleSnap works.
_______________________________
9:13 AM
_______________________________
9:13 AM
Introducing StyleSnap, feature on the Amazon App that lets customers shop for apparel by taking a screenshot of a look or style they like.
_______________________________
9:12 AM
_______________________________
9:12 AM
People don’t shop the way they did when Amazon started in 1995, or even the way they did in 2015. We started as a bookstore. We are now a collection of Amazon Stores.
_______________________________
9:11 AM
_______________________________
9:11 AM
We're going to focus on three areas where we’re deploying AI to shape customer experiences in Shopping, Delivery, and Voice.
_______________________________
9:11 AM
_______________________________
9:11 AM
As we developed AI technologies across Amazon, we didn’t sequester our AI scientists in their own group. Instead, we placed them in our businesses, integrating scientists with the folks building the products, and we started with the customers and worked backwards.
_______________________________
9:10 AM
_______________________________
9:10 AM
In bettering the algorithm for customers, the teams introduced a two-layer neural network called a Neural Network Classifier.
Using Prime Video as an example, "This model was completely focused on predicting what customers wanted to watch in the next week. We took historical movies that a customer watched and then asked the model to predict the movie a customer would watch in the next week."
_______________________________
9:07 AM
_______________________________
9:07 AM
On product discovery
The team used"collaborative filtering" (a machine learning model invented by Amazon) to drive our product recommendation engine in the early days. Then combined it with heuristics to develop personal recommendations.
_______________________________
9:02 AM
_______________________________
9:02 AM
Some of us have been working with AI for decades, but we are only in the beginning stages of understanding how AI is improving our lives.
Jeff Wilke - CEO worldwide consumer Amazon
_______________________________
9:01 AM
9:01 AM
Jeff Wilke has taken the stage, welcoming the crowd and opens with AI conversation. In his role, his teams include Prime, Robotics, Fulfillment, Amazon Business, Amazon Go, Whole Foods, Prime Air, and, of course, Amazon.com, Amazon.de, Amazon.co.jp, and more. Together, these groups account for nearly 600,000 Amazonians.
_______________________________
8:57 AM
_______________________________
8:57 AM
We're almost ready for the keynote, backstage now.
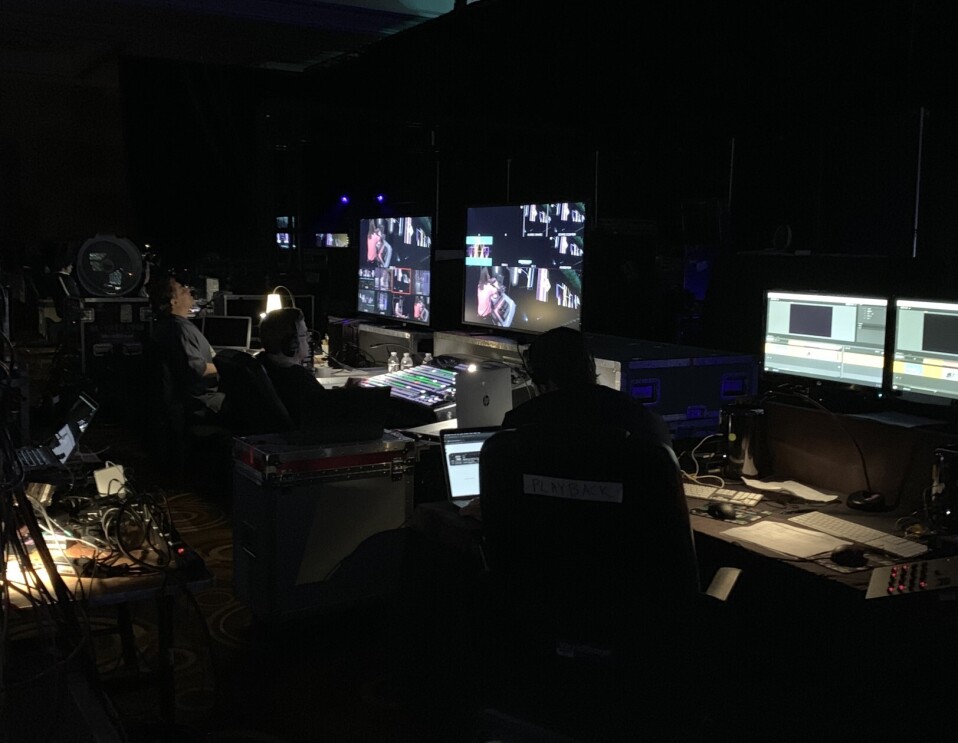
_______________________________
8:07 AM
6/5/2019
8:07 AM
6/5/2019
We're back with the second day of re:MARS. Coming up at 9am is a keynote by Jeff Wilke, CEO worldwide consumer, Dilip Kumar, Vice President of Amazon Go, Jenny Freshwater, director of forecasting, Brad Porter, VP and distinguished engineer of robotics, Rohit Prasad, VP and head scientist, Alexa. We'll be sharing details as they happen.
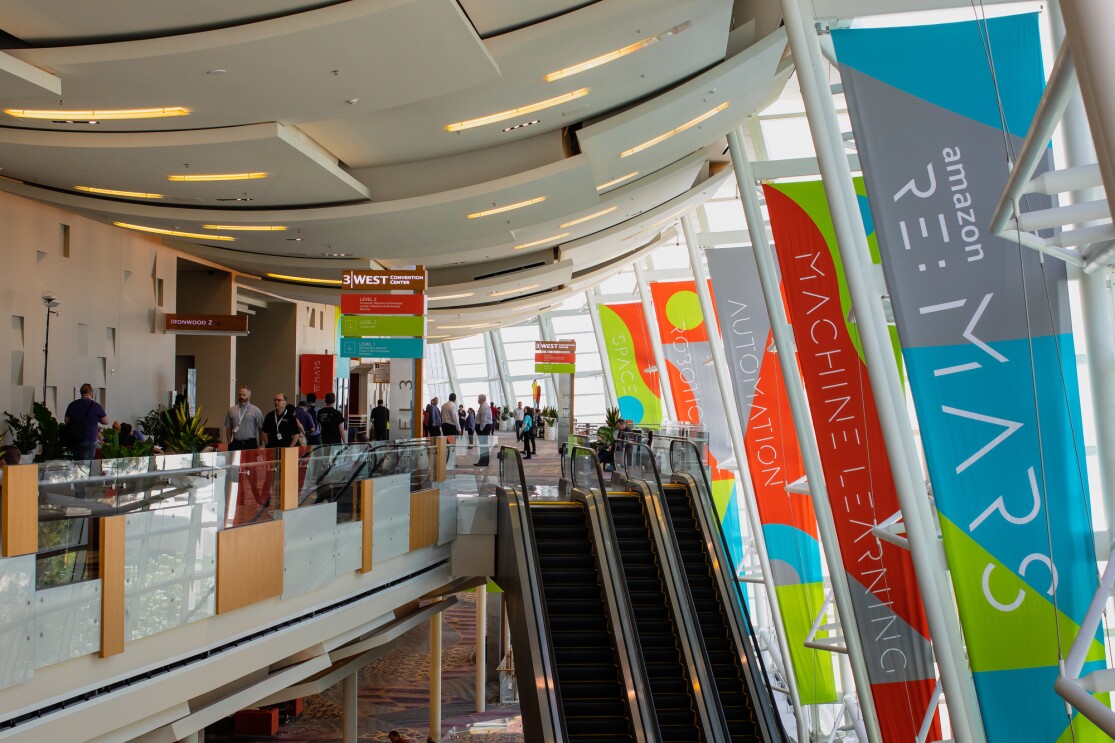
_______________________________
9:30 PM
9:30 PM
That's a wrap for the first day of re:MARS, we'll be back in the morning with the can't-miss keynote, details from breakout sessions on topics such as "Fighting human trafficking using machine learning," "Intentional technology," "Unlocking the creativity of humans," and more.
Sights and activities from the first day at re:MARS.
01 / 08
_______________________________
9:06 PM
9:06 PM
See the opening remarks from Dave Limp, SVP devices at Amazon.
_______________________________
7:53 PM
7:53 PM
_______________________________
6:46 PM
6:46 PM
_______________________________
6:39 PM
6:39 PM
_______________________________
6:21 PM
6:21 PM
Robert Downey Jr. has stepped on stage.
_______________________________
10:05 AM
_______________________________
10:05 AM
_______________________________
6:17 PM
6:17 PM
While we're learning about robots from Disney Imagineering, take a look at a next generation robot at Amazon and the process behind developing it.
_______________________________
6:09 PM
6:09 PM
_______________________________
6:05 PM
6:05 PM
Boston Dynamic's Spot robot went for a walk backstage at re:MARS.
_______________________________
6:03 PM
_______________________________
6:03 PM
Morgan Pope, Associate research scientist and Tony Dohi, Principal R&D Imagineer, both from Walt Disney Imagineering, have taken the stage.
_______________________________
6:02 PM
_______________________________
6:02 PM
_______________________________
5:56 PM
5:56 PM
_______________________________
5:53 PM
5:53 PM
The team is demonstrating two different robots on stage. The robots will be in the Tech Showcase later, so we'll get a chance to see them up close then.
_______________________________
5:51 PM
_______________________________
5:51 PM
Raibert is talking about three different robots today - Handle, Spot, and Atlas.
_______________________________
5:43 PM
_______________________________
5:43 PM
"This is the backdrop for re:MARS – we wanted to bring the spirit of MARS – the combination of builders and dreamers – to a broader group of leaders here in this room. We set out to bring together innovative minds from diverse backgrounds – from business leaders to technical builders to startup founders, venture capitalists, artists, astronauts and more. Our goal with re:MARS is to bring together the right content, learning opportunities, and leaders to help you disrupt and innovate faster – to combine the latest in forward-looking science with practical applications that will initiate change today. We believe that together we can solve some of the world’s most interesting and challenging problems using AI."
_______________________________
5:34 PM
_______________________________
5:34 PM
"The heritage of MARS -- Four years ago, we hosted the first annual MARS conference focused on Machine learning, Automation, Robotics, and Space. MARS is an intimate event designed to bring together innovative minds to share new ideas across these rapidly advancing domains.
On the first night of the very first MARS event, I was talking to Jeff about the event’s inspiration, and we started talking about the library in his house. In Jeff’s library, there are two fireplaces that face each other. On one side of the library, over the fireplace, he has the word Builders, and under that is all of the books in his collection that are authored by builders. And on the other side of the library, he has books by Dreamers. This is a very good representation of what we are trying to do here – to bring together the builders and the dreamers – as we envision the future."
_______________________________
5:33 PM
_______________________________
5:33 PM
"We have an incredibly diverse group here. Our attendees include astronauts, CEOs, artists, entrepreneurs, PhDs, politicians, engineers, business leaders and many more.
We have attendees joining us from 46 countries, with especially large contingents from the US, Canada, China, Australia, Brazil, Germany, Japan, Korea, and the UK.
Here are a few fun facts about some of our attendees, they've...
Spent a combined 562 days in space, including the NASA record holder for most consecutive days in space and most total days in space.
People who invented early applications of the internet and robotics, including the first robot with a web interface that enabled remote visitors to water a living garden via the internet in 1994
Founded companies, including Coursera, iRobot, Insitro, SmugMug, Humatics, Ring, and more
And even have won a Golden Globe, an Olympic gold medal and 5 NBA championships"
_______________________________
5:31 PM
5:31 PM
Dave Limp has taken the stage.
"Over the course of re:MARS, we're excited for attendees to spend the upcoming days at workshops by training a neural network for computer vision to win at simulated Blackjack, building a Martian-detecting robot application and more."
_______________________________
5:21 PM
5:21 PM
DJ booth before the keynote.
_______________________________
4:31 PM
4:31 PM
One hour from now, Dave Limp will open the keynote, with spotlight talks from Marc Raibert, CEO of Boston Dynamics, Morgan Pope and Tony Dohi of Walt Disney Imagineering, and Robert Downey Jr.
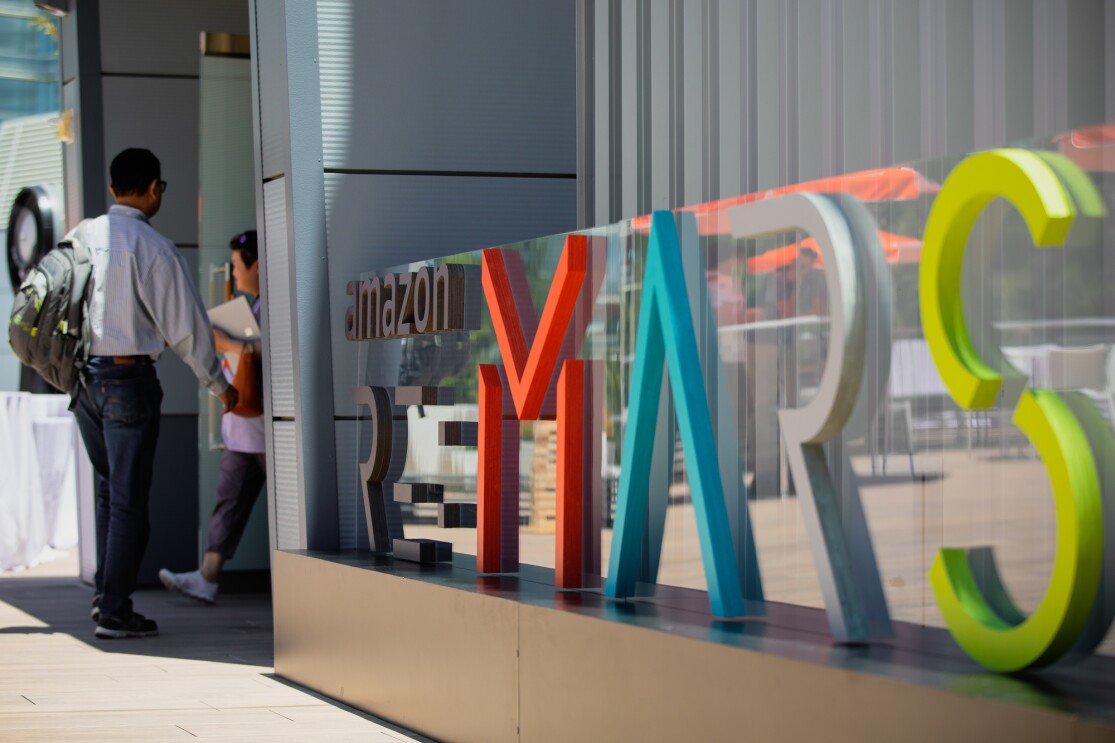
_______________________________
4:10 PM
4:10 PM
re:MARS Blackjack Challenge
Teams use computer vision and machine learning to build and train a neural network for computer vision using Amazon SageMaker, then develop an algoithm to try to win against the house. Each of the teams participating will get a chance to try their algorithm out.
01 / 02
_______________________________
3:39 PM
3:39 PM
DJ spinning beats outside the registration hall.
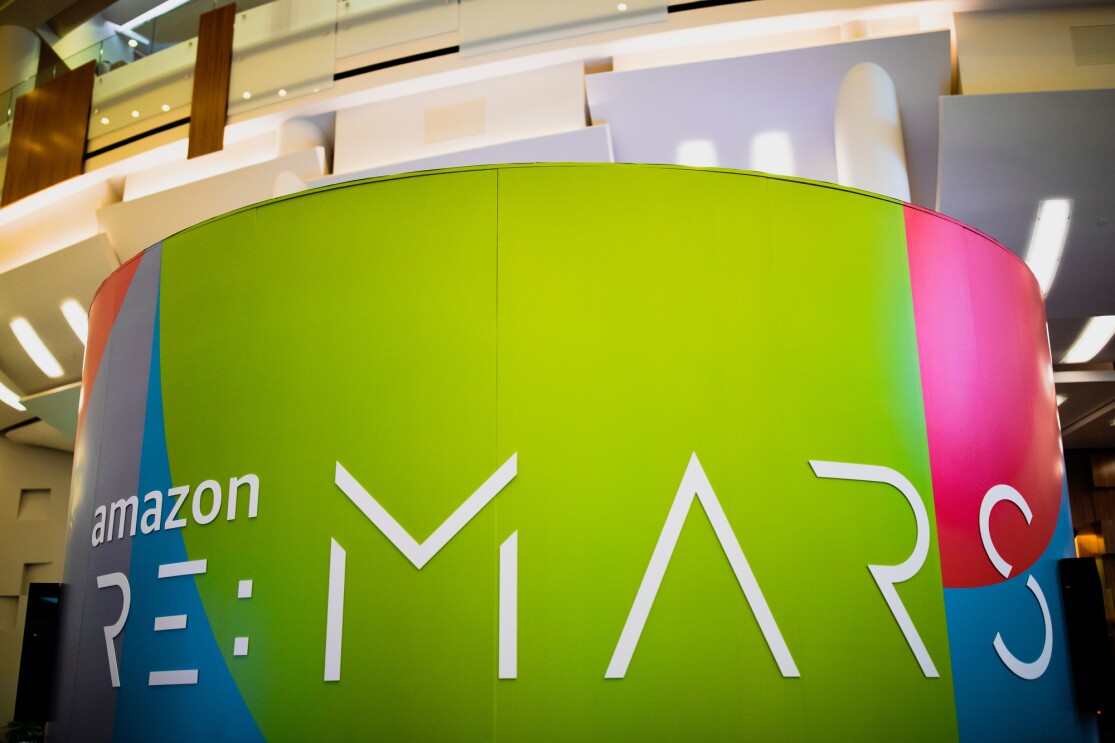
_______________________________
2:39 PM
2:39 PM
_______________________________
2:13 PM
2:13 PM
At the “Smart cities are the future session” at re: MARS, attendees are using the city of Las Vegas Open Checkbook data portal to build a voice application with Alexa, and ask Alexa for answers to questions like, “Which department did we spend the most on in 2019?” The future of our cities is interactive, and the future is now.
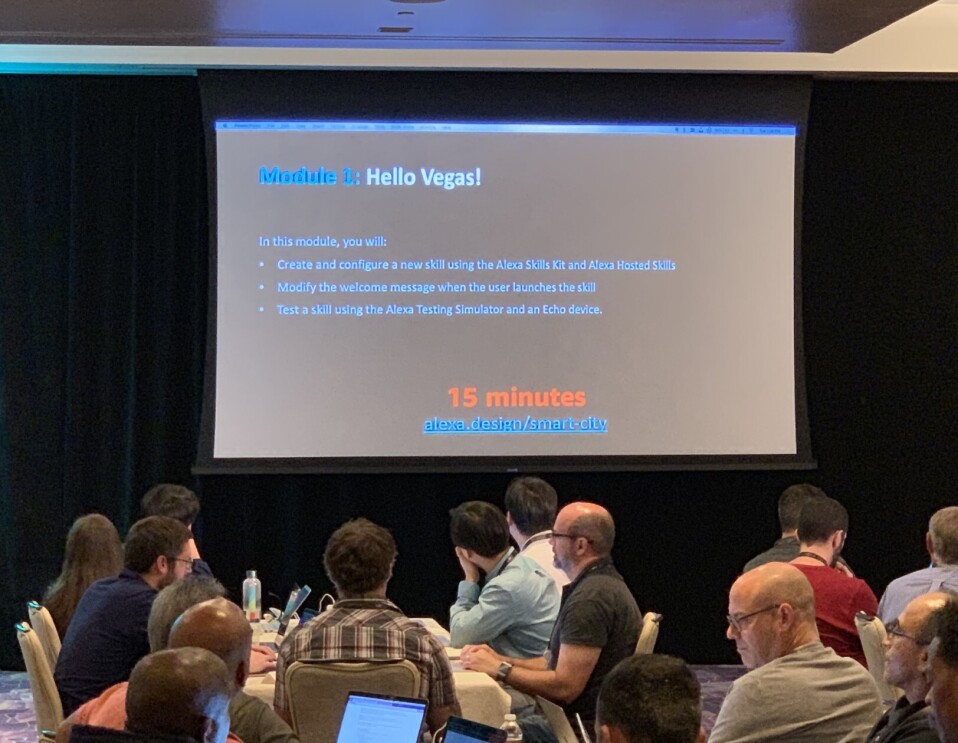
_______________________________
1:27 PM
1:27 PM
Allan MacInnis, AWS solutions architect is leading "Finding Martians with AWS RoboMaker and the JPL Open Source Rover" session.
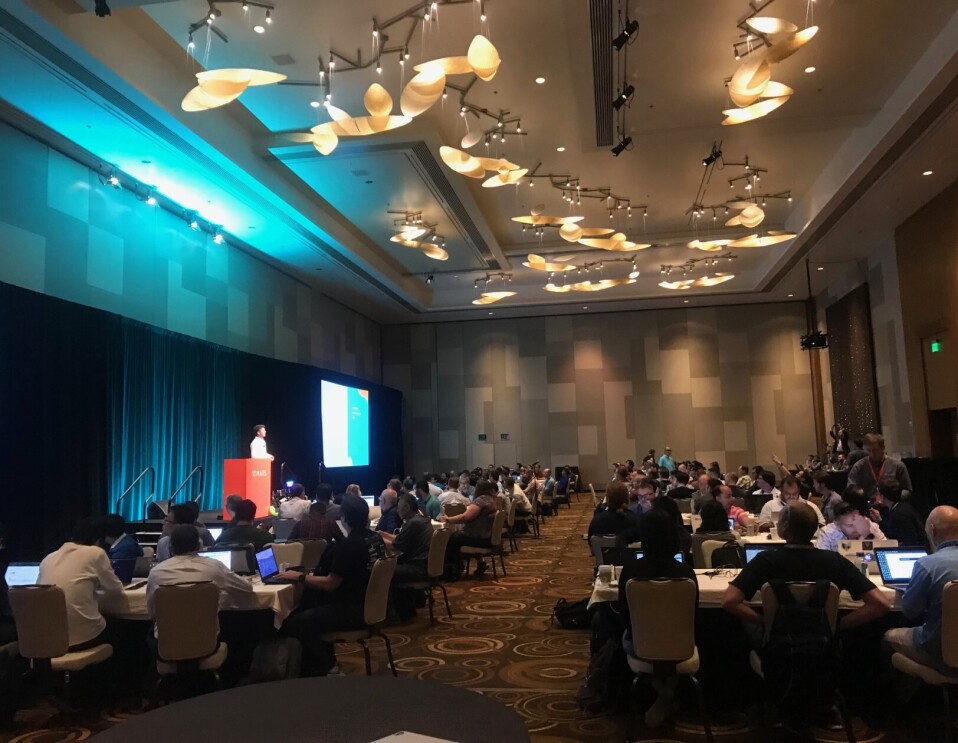
_______________________________
1:01 PM
1:01 PM
Next up: hands-on workshops are starting now. We're digging into "Finding Martians with AWS RoboMaker and the JPL Open Source Rover," "Smart cities are the future," and "re:Vegas Blackjack." Find out more.
_______________________________
12:37 PM
_______________________________
12:37 PM
Did we mention that there will be BattleBots, on Thursday?
_______________________________
12:14 PM
12:14 PM
Robert Downey Jr., Marc Raibert - CEO, Boston Dynamics, Morgan Pope - Associate research scientist, Walt Disney Imagineering, Tony Dohi - Principle R&D at Walt Disney Imagineering, will be speaking later today. Stay tuned for quotes, product news, future thinking, and more.
_______________________________
10:45 AM
10:45 AM
Walking toward registration, it's apparent we're at re:MARS. Behind the curved wall is the Blue Origin capsule (see the video below). Beyond that are the registration queues, and a swag booth.
_______________________________
9:40 AM
9:40 AM
Of course we have a Blue Origin capsule on-site. Take a look inside.
_______________________________
9:19 AM
6/4/19
9:19 AM
6/4/19
It's the first official day of re:MARS - we'll be sharing an behind the scenes peek from the event - with everything from cool new products and announcements, to thought leadership in machine learning, robotics, artificial intelligence, space, and more.
_______________________________
5:41 PM
_______________________________
5:41 PM
It wouldn't be a conference in Vegas without a little blackjack. We're doing things a little differently, challenging teams to use computer vision and machine learning to build and train a neural network to win.
_______________________________
1:25 PM
1:25 PM
Workshops begin at 9am tomorrow, and registration opens at 7am. See , now.
_______________________________
7:29 AM
6/3/19
_______________________________
7:29 AM
6/3/19
Attending re:MARS? Here's what to expect from the #reMARS party - and there's so much more going on.
Watching from home? We'll be sharing details about the party, keynotes, sessions, speakers, and more, right here.
_______________________________
10:19 PM
6/2/19
10:19 PM
6/2/19
re:MARS officially begins in less than 36 hours, and we are ready for it. Stay tuned for live updates, a behind-the-scenes peek, robots, drones, breaking news, and more.