Nature does not move in straight lines. Just look at the image below.
What you see is a Sentinel-1 radar satellite image taken above the Andaman Sea off the coast of Thailand. What is that dark arrow-shaped line?
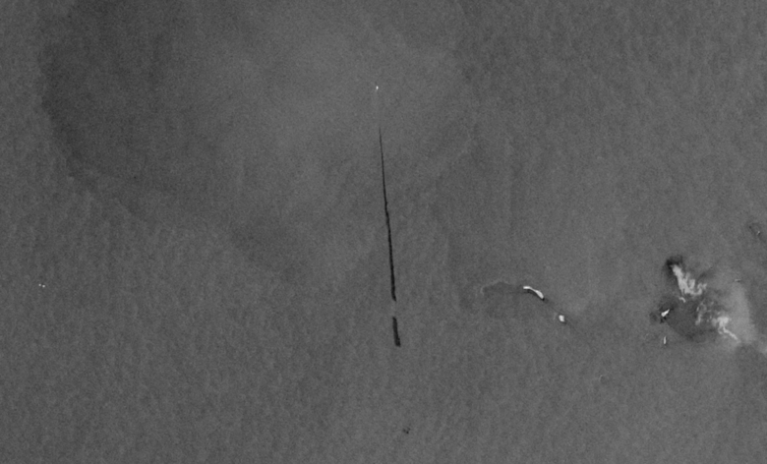
The image was taken in the middle of a highly trafficked sea, so a good guess is the line is a ship’s wake, which is partially correct. But there’s also something illegal.
Rather than just churning up the seawater, this ship is dumping its oily wastewater—or bilge water—into its wake. Bilge water contains a toxic combination of oil, lubricants, grease, cleaning fluids, and a long list of other harmful metals and chemicals. The image shows how the slick follows the direction of the ship’s travel, starting from the bottom of the image at the dark line’s widest point, where the dumping began, and extending to where it narrows to a point as the ship empties its bilge.
“You can’t unsee that now,” said John Amos, president of SkyTruth, a nonprofit that aims to use satellite imagery to inspire people to protect the environment. “We're motivated to take environmental issues that are flying under the radar for most people, like illegal bilge water dumping, and literally put them in front of people with a picture so they can see it.”
SkyTruth hopes to sear those images into the minds of policy makers, politicians, corporate boards, and others who can make a difference so that they’ll take action to address the environmental issues we face worldwide. For Amos and the SkyTruth team, that includes revealing how oil slicks are polluting the oceans and how commercial fishing activity is depleting fish populations. The nonprofit also wants to show how mining practices are fouling rivers and destroying landscapes in the Appalachia region of the eastern United States and in the Amazon rainforest of South America.
With satellite imagery increasingly available to the public (the AWS Open Data Sponsorship Program hosts Copernicus Sentinel-1 radar data), SkyTruth is able to now show the world what used to be hidden from public view.
“As big data technologies and cloud computing have come into being, we have rushed to take advantage of those technologies to scale our ability to tell those stories and to confront the public with these images,” Amos said. “And we've started to make pictures with data.”
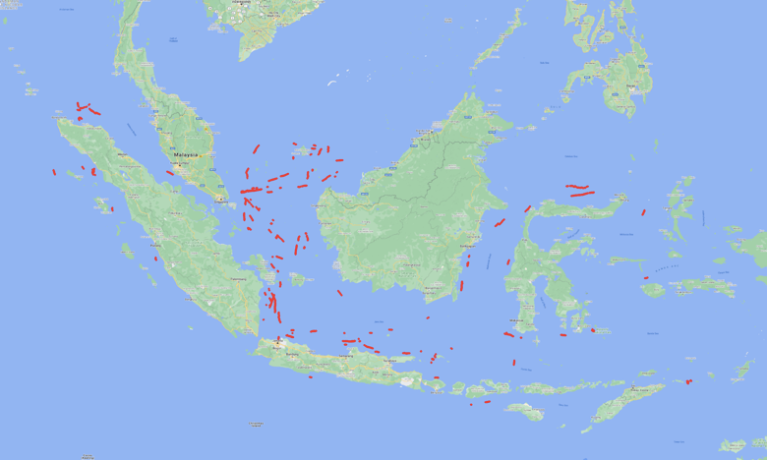
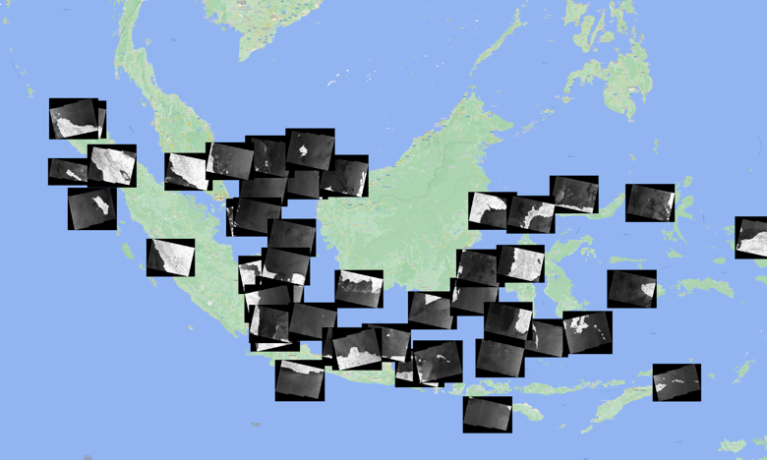
The idea to tackle oil slicks came after SkyTruth helped establish Global Fishing Watch, a platform that uses satellite data to reveal fishing activity throughout the ocean. Amos said he learned his way around satellite imagery while working as a geologist.
“I'd seen repeated imagery showing these unexplained oil slicks out in the ocean,” Amos said. “It’s like, ‘hey, there's no oil platforms out there, and there is this straight-as-a-ruler trail in the water.’ So what is it, and what is causing it? Turns out, when civilian radar imaging systems came into being, like Sentinel-1, there was the opportunity to do a lot more of that because radar turns out to be the ideal tool for detecting oil slicks on the water.”
The SkyTruth team had the imagery but was missing the people-power required to examine countless images of the world’s oceans to look for the tell-tale lines that indicate a passing ship had created an oil slick. The team figured out that machine learning—specifically a well-trained “deep neural network” model—was particularly well suited for finding the distinctive lines.
Deep learning is an approach in machine learning in which a model is trained to “learn” something, such as recognizing a sound or speech, like when you ask Alexa to play your favorite song. Deep learning models are powerful multi-purpose tools that can also recognize images, identify what sport you’re playing based on the motion of a phone in your pocket, or generate movie scripts from a single prompt. The more training a deep learning model receives, the more accurate it becomes. In the case of bilge water dumping, the deep learning model works to identify the specific characteristics of an oil slick in a satellite image—and not something of a similar shape in the ocean.
SkyTruth began building a machine learning model to spot oil slicks as part of the2020 AWS Imagine Grant winner cohort. Dubbed “Cerulean,” the effort is SkyTruth’s answer to how a small nonprofit can use machine learning to manage what was effectively an insurmountable amount of data.
Thousands of new radar satellite images are made available in the cloud every day, and hundreds of those images cover parts of the ocean. Over the next few years, as more satellites are put into orbit, tens of thousands of new ocean images could come in daily. Downloading and manually looking at those images would require an army of trained interns or volunteers. SkyTruth does not have such an army—but it does have Jona Raphael, Cerulean’s chief developer and a machine learning expert.
Raphael is training the model to identify the tell-tale signs of an oil-slick in images. One of his toughest obstacles is training the model not to confuse oil slicks with other things in the ocean that can mimic the signature of an oil slick, such as naturally occurring algae blooms or an upwelling of oil from an undersea seep or shipwreck.
“This detection technique relies on the fact that the band of radar that these satellites use for imaging, the C-band, is very sensitive to the roughness of the ocean surface,” Raphael said.
At a wavelength of about 5 centimeters, the C-band is roughly the same wavelength and height as the ripples on an unspoiled ocean surface when the wind is blowing. An oil slick prevents such ripples, meaning the radar can detect oil-caused flat areas on the ocean’s surface.
“The wind can't kick up those ripples when there's oil on the water,” Amos said. “The oil is actually slippery. The surface tension, the mechanical connection between the wind and the water is less than in a natural state. So the wind doesn't have as much ability to rough up the surface of the ocean.”
In other words, a stretch of ocean fouled by oil looks smooth and black in the radar satellite image because the incoming radar energy bounces off the oil rather than being scattered back at the satellite by ripples in the water. By detecting unnaturally smooth water in a straight line, the deep learning model detects what it believes is an oil slick.
Currently, more than half of the images Cerulean’s deep learning model identifies are indeed oil slicks. But the beauty of deep learning models is that they learn. So as the model analyzes more images, it will become more accurate. In 2020, Cerulean correctly identified 130 oily bilge water dumping events each month, but accounting for limited coverage of the world’s oceans, that figure suggests the actual number is closer to 800 per month, according to the SkyTruth team. The observed slicks predominantly plague the oceans of Southeast Asia, parts of the African coast, and the Eastern Mediterranean.
“Somewhere between an 80 to 90% true positive rate will be really lovely, and we’ll get there,” Raphael said. “And once we can nail that down, I think that's when we open the doors and share all of this with the outside world.”
That underlies SkyTruth’s mission: to show the world what it hasn’t seen before and prompt change. In the case of oil slicks, that includes identifying specific vessels dumping their bilge water, based on time and location, to hold them accountable and deter vessels from dumping in the first place. It could also mean spotting oil leaks at offshore rigs before they have major environmental impacts.
The power is evident in the approach the small team is taking. They have access to satellite image data, with much more coming. And they hope to develop all kinds of machine learning models to tackle all kinds of problems.
“We dream about building an image-handling pipeline, like the one Jona has built for Cerulean, but then running multiple models against the same image data stream,” Amos said. “You've got one model that's detecting oil pollution. You've got another model that's just looking for vessels in the ocean. We've got another model that's looking for gold-dredging boats on Amazonian rivers. And then you've got another one that's looking at deforestation events in the rainforest.
“One data set, and you can throw as many models at it is as you want to because it's hosted in the cloud. You just identify the images you're interested in, pull them out, and run an analysis on them. Then you share them with the world. If you can see it, you can change it.”