“Data scientist” might be a less exotic job title than it was a few years ago, but that doesn’t mean everyone understands exactly what the role involves or whether a career in the field could be for them.
Most organizations now generate, accumulate, and store massive amounts of data; however, many of them don’t know how to use all the information they’ve gathered to its fullest potential. This is where data scientists come in. Amazon Web Services (AWS) data scientists are simultaneously innovative researchers and skilled storytellers—revealing the trends hidden deep in these big data sets, which can help transform our customers’ businesses.
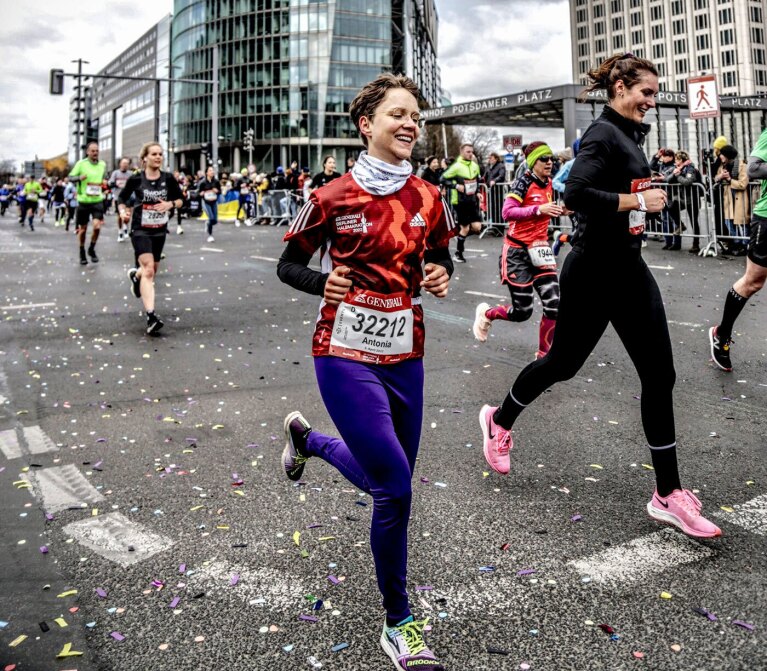
Antonia Schulze is a data scientist based in Berlin, Germany, in the AWS Machine Learning (ML) Solutions Lab. We spoke to her about some of the projects she’s worked on, what ML looks like in the real world, and her tips for pursuing a career in data science—regardless of whether you have a traditional skill set in the field.
Tell us about your role at AWS
My job involves analyzing a large amount of data and deriving valuable insights from it, using a mix of business acumen and statistics, as well as ML techniques. I usually describe ML to people who aren’t familiar with it as building systems that can learn from data, without being explicitly programmed by a human. At AWS, I’m involved in helping some of our biggest customers accelerate the adoption of ML in their business. We begin by looking at their vision and finding the highest-value use cases for ML. We then run short pilot projects—or “proof of concepts”—using the latest ML models to solve the specific problem.
For a manufacturing customer, ML might be used to improve research and development or supply chain functions. For example, we’ve helped manufacturing companies more accurately detect defects and reduce the time employees spend doing manual inspections by half. In the automotive industry, data can be used to help improve the safety of drivers and pedestrians, such as in the development of autonomous vehicles. Data and advanced analytics can create more advanced road scene perception, which helps autonomous vehicles to see their surroundings accurately and assess any risks.
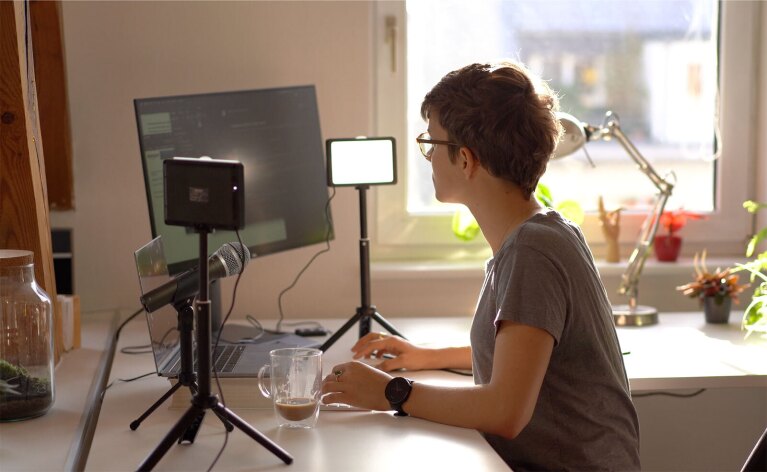
Where can we see AWS ML in action?
ML is everywhere today. A great everyday example is in sports. In the U.S., the NFL uses AWS ML technology for its “Next Gen Stats”—an advanced analytics platform that brings fans exciting new insights on the game of American football. Our ML models track an array of complex data sets coming from the field to produce stats on everything from the fastest ball carriers to the probability of a completed pass. Meanwhile, in Formula 1 (F1), “F1 Insights” powered by AWS gives fans a better understanding of everything from the fastest drivers in the sport’s history to how drivers attempt a perfect start, their braking performance, probability of completing an overtake, and much more.
These kinds of data-driven insights provide viewers with more meaningful information, help spark conversation, deepen fans’ knowledge, and ultimately bring people closer to their favorite sport. It’s all about creating a better experience—something that became even more important during the pandemic, when fans couldn't attend sporting events in person.
Do you have a favorite project you've worked on?
During the earlier stages of the pandemic, we worked directly with the World Health Organization (WHO) on a project tackling the wave of misinformation and “fake news” about COVID-19. The challenge for WHO was the volume of information that needed analyzing, as there was more than there had ever been previously. We helped WHO develop and incorporate ML models into their public health tools to analyze and classify large volumes of COVID-19-related content from around the world. The ML models we helped develop can assist WHO analysts assess the trustworthiness of information and make credible sources easier to find and analyze. This type of work sits within the field of natural language processing (NLP), a key area in artificial intelligence (AI) and ML. It was a unique project because we had to understand how to handle entirely new data sets with no precedent for the scale or speed required.
What’s the best part of your job?
I love the variety of my work. I’ve already worked with organizations in sports, health care, and more, and I have flexibility to work in other sectors, such as automotive, manufacturing, retail, or any other industry, really. The diverse nature of our work also means we come into contact with people from across the AWS business, from senior leaders to subject matter experts—all of whom are willing to share their time and experience. I love the diversity of thought, personality, and expertise. Someone who is specialized in finance, for example, will work and communicate with you in a different way than someone working in the sports industry. To me, the people I work with—customers and colleagues—are the most interesting part of my job.
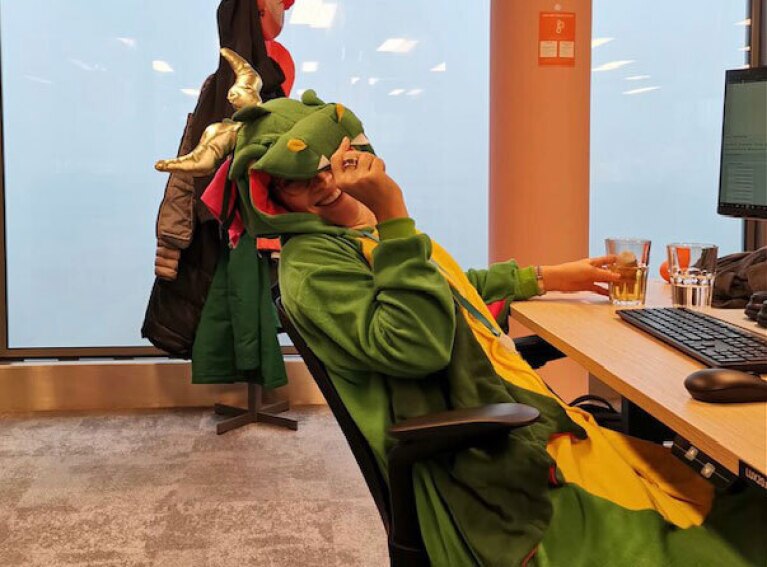
How did you get into data science?
My career journey is a little unconventional. I didn’t study math or science, and unlike many of my colleagues, I don’t have a Ph.D. I wanted to go into business administration at first, so my background is in business and communications. However, I soon realized that I wanted to work in a more technology-focused and data-driven field. I did a market research internship in the technology and media telecommunications sector. This inspired me to learn more about machine learning and data science. I decided to go back to university and study for a master’s in big data and business analytics, which I loved because it focused a lot on customer experience. After that, I initially found it hard to secure a data science position because companies usually require a background in math or statistics.
When I saw that Amazon was hiring interns in business intelligence data science, I applied right away. I went through the recruitment process and got a job on the customer experience team in Amazon Operations. It was really interesting, but not the exact area I wanted to be in. While I was there, I found a mentor working in data science at Amazon, and my manager helped me to look out for the right opportunity within the business. With their support, I was able to approach a hiring manager for a data science role and landed my current job.
What’s the most challenging part of your job?
We’re a very innovative team, so we’re often applying new ideas, tools, and processes to problems that have never been solved before. The most challenging, but also rewarding, part for me is creating an ML model from scratch, especially as my academic background is not in math or statistics. I make sure I’m constantly deepening my knowledge. This might be by developing my network in areas where I have less experience—such as finding a NLP expert to have regular sync-ups with—or reading a lot of research papers to help me understand algorithms better.
Mentoring and support go both ways. When somebody is generous with their time, I always want to provide value in return. With my business and communications background, one way I can help is to translate complex, scientific explanations into accessible, user-friendly language. That might be communicating the results of a data science project to customers who may not have a technical background, or sharing news about our innovations internally with Amazon employees across the business.
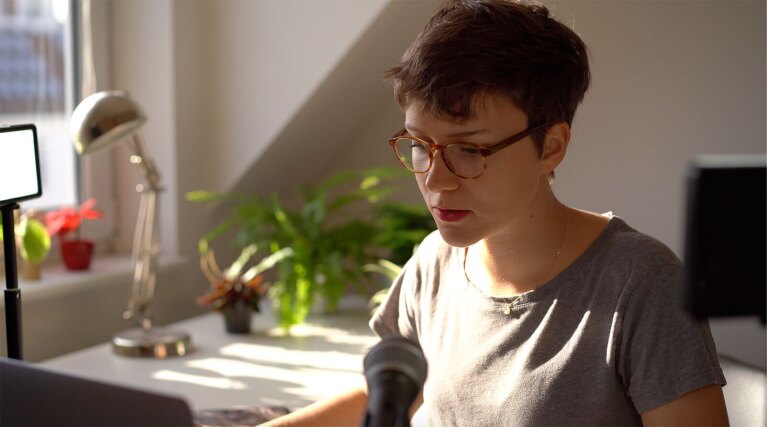
How would you like to see ML and data science evolve?
We need more women in leadership roles, and for them to be visible, and honest and open about some of the challenges that they face. I founded the Women in ML/AI global chapter of the Women@ affinity group at Amazon last year. Women interested in working in this area may have the same problem I did—not knowing where to go for information or what options might exist for transitioning to a data science role without specific qualifications.
The group is open to all Amazon employees. You don’t have to be a woman or working in ML to be part of the community. Most of our women members aren't in ML roles but are looking for support on how to move to one. Through the group, we regularly assess our members’ needs, then we address these with specific events, mentorship programs, and other solutions. For me, greater diversity is key—for AWS and for the future of data science and ML as a whole. A diverse team means diverse perspectives, which in turn can only mean more opportunities to understand your customers better.
AWS is committed to providing opportunities for students and recent graduates from all backgrounds to cultivate their passions and sharpen their skills. Find out more about our internship program.